The University of Toronto have form when it comes to using machine learning in drug discovery. In a recent paper they describe the use of machine learning to generate 3D structures of protein molecules to assist with drug development.
“Designing successful drugs is like solving a puzzle,” the team say. “Without knowing the three-dimensional shape of a protein, it would be like trying to solve that puzzle with a blindfold on.”
They’re putting such thoughts into practice via a spin-out company called Deep Genomics, which was founded by Professor Brendan Frey. The company uses deep learning to trawl through genetic data to try and identify the genes responsible for specific diseases so that medicines can be created.
Detecting mutations
To date, the company has primarily focused on exploring the genome for mutations, which whilst difficult to detect can play a key role in particular diseases. For instance, an early target is to hunt for genes associated with Mendelian disorders whereby a single genetic mutation plays a significant role.
As both the data available has mushroomed, through both lower cost genetic sequencing but also more lifestyle data, and computational power has grown in sync to enable this big data to be analyzed efficiently, it has led to a significant increase in interest in computational approaches to medical research.
One such is Spanish bioinformatics startup Mind the Byte. They aim to help clients perform in silico drug development analysis via their SaaS platform. The pay-per-use software aims to take many of the costs associated with such work out, thus making it more accessible for small and medium sized biotech companies.
Or you have fellow Spanish venture Naru, who attempt to personalize biomedicine by combining genetic data with medical records and qualify of life measurements via statistical analysis. As such, they hope to be as useful in clinical practice as in clinical trials.
This points to the tremendous promise of such approaches, both in working with enormous data sets that comprise genetic and lifestyle health data, but also with very small pathology data sets.
Carnegie Mellon researchers famously suggested that drug development costs could be cut by 70% with better use of automation, and with the development of medicines still usually very lengthy, very costly and very risky, it’s a process that is ripe for disruption. We’re still at a very early stage, but the promise is considerable, and it will be fascinating to see just where things go from here.

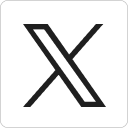



