AI is increasingly being deployed in drug development, with a range of approaches used to identify molecules, assess drug toxicity and so on. The University of Toronto are at the forefront of this movement, and a team of researchers from the university have recently released a study highlighting how their deep learning algorithm was used to track proteins.
The project aims to use AI to understand more about what exactly makes cells healthy, and how they fail as disease strikes.
“We can learn so much by looking at images of cells: how does the protein look under normal conditions and do they look different in cells that carry genetic mutations or when we expose cells to drugs or other chemical reagents? People have tried to manually assess what’s going on with their data but that takes a lot of time,” the team say.
The system, known as DeepLoc, uses an algorithm to spot patterns in the cell made by proteins, with the team confident that their approach is considerably faster than both humans and existing computer-based approaches.
Augmented cell biology
AI has had an impact on a great many fields, but the potential for cell biology is particularly strong. The latest generation of microscopes are fully automated, thus providing scientists with huge amounts of data. The challenge now is how to analyze it efficiently.
“Right now, it only takes days to weeks to acquire images of cells and months to years to analyze them. Deep learning will ultimately bring the timescale of this analysis down to the same timescale as the experiments,” the team say.
The project trained the algorithm on a huge number of images of glowing proteins inside cells. What made the approach interesting however was that it required no human input for the algorithm to learn, thus making it considerably faster, but also more accurate. The image library had been previously used by a prior iteration of the computer vision software, but whilst that software took months to crunch the data, DeepLoc was able to get through it in hours.
As with many AI based vision analysis projects, DeepLoc was able to spot subtle differences between images that ostensibly look identical. The initial analysis identified 15 different classes of proteins, each representing distinct neighbourhoods in the cell; DeepLoc identified 22 classes. It was also able to sort cells whose shape changed due to a hormone treatment, a task that the previous pipeline couldn’t complete.
“Someone with some coding experience could implement our method. All they would have to do is feed in the image training set that we’ve provided and supplement this with their own data. It takes only an hour or less to retrain DeepLoc and then begin your analysis,” the authors say.
The team have developed a startup to help commercialize the technology. The venture, called Phenomic AI, hopes to work with pharmaceutical companies in analyzing cell image-based data.
“In an image based drug screen, you can actually figure out how the drugs are affecting different cells based on how they look rather than some simplified parameters such as live/dead or cell size. This way you can extract a lot more information about cell state form these screens. We hope to make the early drug discovery process all the more accurate by finding more subtle effects of chemical compounds,” they say.

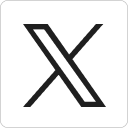



