Autonomous vehicles traditionally require billions of miles under their wheels before they can swiftly and safely respond to the diversity of conditions they may face. A team from the University of Michigan believe they may have found a way to short-cut that process by as much as 99%.
In a recently published paper by the Mcity partnership that the university lead, they outline the approach in more detail.
“Even the most advanced and largest-scale efforts to test automated vehicles today fall woefully short of what is needed to thoroughly test these robotic cars,” the authors say.
Modular driving
The team utilize a modular approach to driving that sees situations broken down into components that can be easily tested repeatedly. This exposes autonomous vehicles to a condensed set of the most challenging driving conditions. It’s an approach that sees 1,000 miles yield the same returns as driving up to 100 million miles in real-world conditions.
This kind of volume is required due to the huge variety of scenarios a car may face. Indeed, fatal crashes on the road typically only occur every 100 million miles or so of driving.
The paper argues that for us to be confident in autonomous vehicles will require cars to be 90% safer than human drivers. It’s a level that they estimate would require something like 11 billion miles to be driven in real-world settings, which would take well over a decade to achieve. The technology will require a fundamentally different form of testing to that currently used today, especially in the event of a crash occurring.
“Test methods for traditionally driven cars are something like having a doctor take a patient’s blood pressure or heart rate, while testing for automated vehicles is more like giving someone an IQ test,” the authors say.
Taking the short cut
The researchers developed their four-step accelerated approach by analyzing data from over 25 million miles of real-world driving undertaken by nearly 3,000 vehicles over a two year period.
By analyzing the data, they were able to identify events that contain meaningful interactions between a human driven car and an automated one. They could then use this to create a simulation that purely contained these meaningful interactions rather than uneventful ones.
They then coded the simulation so that it considered human drivers as the primary threat to autonomous vehicles, with human drivers placed randomly throughout the simulation.
The team then used maths to assess the risk and probability of various outcomes, such as crashes and near-misses, before using importance sampling to assess the test results.
They believe that this rapid form of evaluation can be effectively used for a range of dangerous maneuvers. The team put it to the test on the most common situations that tend to result in crashes (usually involving interaction between human and autonomous drivers), and the robustness was good, although they admit that more work is required to test it over a wider range of scenarios.

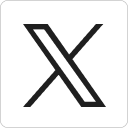



