Sepsis is one of those issues that is largely unreported when it comes to serious healthcare problems, yet it is believed to cost nearly £8bn a year in the UK alone. A more effective method of identifying the risk of sepsis therefore could be very valuable, and that is exactly what researchers from the University of Pennsylvania have come up with.
In a recently published paper, they describe how their machine-learning algorithm is capable of identifying patients at risk of developing sepsis in hospital from nothing more than their electronic health records (EHRs).
“We have developed and validated the first machine-learning algorithm to predict severe sepsis and septic shock in a large academic multi-hospital healthcare system,” the authors say. “This is a breakthrough in the use of machine learning technology, and could change the paradigm in early intervention in sepsis.”
Artificial help
To try and help matters, the researchers turned to machine-learning to try and predict the patients most at risk of developing severe sepsis, with staff then being given such information to make earlier interventions.
The algorithm itself was trained using a random forest classifier to understand data from over 162,000 patient records from people discharged from three University of Pennsylvania Health System acute care hospitals over a three year period.
Once trained, the algorithm was able to continuously monitor hundreds of different variables in real-time to hunt for signs of severe sepsis in patients. The algorithm was capable of detecting signs of severe sepsis 12 hours before the actual onset (as determined by both lab results and physiological data).
The system was tested over a 3 month period on over 10,000 patients as they were being cared for in the three hospitals used in the study. During that time, 3% of all acute care patients were identified as being at risk of developing sepsis, with 10 alerts sent to staff every day.
“We were hoping to identify severe sepsis or sepic shock when it was early enough to intervene and before any deterioration started,” the researchers say. “The algorithm was able to do this. This is a breakthrough in showing that machine learning can accurately identify those at risk of severe sepsis and septic shock.”
The next steps for the project are to examine the way the algorithm can be used in real hospital environments, and how processes may need to be modified to fully capitalize on the system.

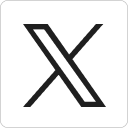



