AI has been fueled by the rise in both data and the computing power to process that data. Its rise has brought understandable concern about how that data is governed by the wide range of actors who have a stake in it. Earlier this year, for instance, the Royal Society issued a report calling for strong and robust data governance to be at the heart of next steps in the development of AI.
It followed hot on the heels of an earlier report by the European Commission that examined the use of big data in healthcare specifically, and the policy implications over the coming years.
It is perhaps no surprise therefore that the UK’s independent data governor The Information Commissioner’s Office have added their voice to the debate via a new guidance paper on Big Data and Data Protection.
The paper, which is an updated version of an original 2014 publication, examines the impact big data, artificial intelligence and machine learning has on data protection.
Modern data protection
“Big data, AI and machine learning are becoming widespread in the public and private sectors. They may increasingly be seen as ‘business as usual’, but the key characteristics of big data analytics still represent a step change in the processing of personal data,” the authors say.
They make a number of recommendations to help organizations adapt their data governance for this modern world.
- Consider whether big data analytics actually requires processing personal data (often it doesn’t). If it does, you should use anonymization where possible.
- Transparency should be a given when processing personal data, with meaningful privacy notices provided at various stages of the big data work.
- Use a privacy impact assessment to identify clear privacy risks. This should have input from all stakeholders in the project.
- Adopt privacy by design in the development of your project, including data security, data minimization and data segregation.
- Develop clear ethical principles to reinforce your data protection principles. In bigger organizations, this should consist of an ethics board to scrutinize projects.
- Ensure that any machine learning algorithms you use are auditable, both internally and externally to ensure that their workings are explainable and as free from bias and discrimination as possible.
In a field that is taking on growing importance, the paper provides a nice contribution to better working in the area.

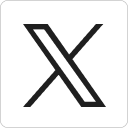



