I’ve written a number of times recently about the prospects for automation and AI to enhance the research and development of new medicines, whether it’s in supporting more effective research, testing for side effects or in the production of drugs themselves.
A recent paper examines this in more detail, with a specific look at how chemical engineers mass produce the chemical compound itself. There can often be 100s of different sequences of reactions that produce the same end result, but some will use cheap reagents than others. Equally, some are easier to run continuously.
Efficient drug production
The team, from MIT, used a machine learning algorithm that was trained using thousands of previous experimental reactions to learn and then predict what a reaction’s main products will be. The system was able to predict the major product of a reaction 72% of the time.
“There’s clearly a lot understood about reactions today,” the authors say, “but it’s a highly evolved, acquired skill to look at a molecule and decide how you’re going to synthesize it from starting materials.”
As with many machine learning applications in healthcare today, the aim is to speed up the process by which that understanding about reactions is obtained. Suffice to say, the algorithm will need to be refined to improve upon its current 72% success rate, but even now the team believe it can help chemical engineers converge on the best sequence of reactions faster than they do today.
Traditionally, chemists have used computer models to characterize the reactions, but even these usually require scientists to research exceptions themselves, with sometimes more than a dozen of these exceptions required for a single model.
Circumventing the process
If nothing else, the team hope to circumvent this process. The system was trained using 15,000 observed reactions recorded in patent filings. It was important for the system to learn what didn’t occur as well as what did, so more training fodder was sought. The team generated a number of additional possible products based on the reaction sites. The team then fed the system descriptions of reactions into the algorithm to rank possible products in order of likelihood.
This allowed the system to form a hierarchy of reactions without requiring any form of human input at all. Overall it provides an interesting approach towards targeted synthesis that a number of drug companies have already expressed an interest in.
“Currently we rely heavily on our own retrosynthetic training, which is aligned with our own personal experiences and augmented with reaction-database search engines,” Novartis say. “This serves us well but often still results in a significant failure rate. Even highly experienced chemists are often surprised. If you were to add up all the cumulative synthesis failures as an industry, this would likely relate to a significant time and cost investment. What if we could improve our success rate?”

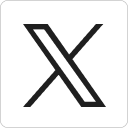



