The development of treatments for Alzheimer’s disease has perhaps not gone as the industry would have liked in recent years, with new drugs still failing to really crack it, despite enhanced understanding of the condition. A recent paper argues that the cause of this slow progress is the undefined subtypes of mild cognitive impairment, which are usually a precursor to Alzheimer’s.
“Everyone thinks Alzheimer’s is one disease, but it’s not,” the authors say. “There are many subgroups. If you enroll all different types of people in a trial, but your drug is targeting only one biological pathway, of course the people who don’t have that abnormality are not going to respond to the drug, and the trial is going to fail.”
The authors believe that if people with similar forms of cognitive impairment are grouped together, when investigational drugs can be tested more precisely.
The team used a multilayer clustering algorithm to sort through dozens of different data points to try and find similar disease types. The data itself came from the Alzheimer’s Disease Neuroimaging Initiative and included cognition tests, brain scane and spinal fluid biomarkers.
Two distinct groups emerged. One of these saw the cognition of participants decline significantly, whilst another where minimal decline was observed. Among the rapidly declining group, their progress from mild cognitive impairment to full-blown dementia was five times faster than their peers in the second group.
The team believe that such an approach could be used to create more homogeneous trial groups, which in turn would allow researchers to design shorter trials whilst still securing strong insights into the potential effectiveness of treatments.
“The findings have direct implications for the design of future trials,” they say. “We have known bits and pieces of this information — that there are dozens of genes that put people at risk, or that certain brain changes put people at risk — but using unbiased tools, such as data mining with this novel algorithm, we can put all of these pieces of information together to identify those who are truly at the greatest risk.”
Data based medicine
Suffice to say, the team are not the only ones looking to utilize big data and AI to detect Alzheimer’s at an earlier stage. Research led by a team from the University of Granada have developed a method that uses high-level data abstractions to allow computers to differentiate between a healthy brain and an ill one automatically.
In a recently published paper, the researchers explain how their machine learning based approach allows them to detect conditions such as Alzheimer’s at an early stage.
The AI enables the researchers to use high-level data abstractions to differentiate between a healthy brain and that of one suffering from a range of conditions, such as Alzheimer’s and Parkinson’s. It does so by automatically extracting the region of the brain affected by the condition.
“The study uses deep learning techniques to calculate brain function predictors and magnetic resonance imaging to prevent Alzheimer’s disease. To do this, we have used different neural networks with which to model each region of the brain to combine them afterwards,” the researchers explain.
Both projects are undoubtedly fascinating, and provide a tantalizing glimpse into the future of medical research.

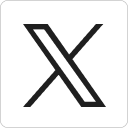



