City planners already receive significant amounts of data about traffic flows, but congestion is still a problem that blights many cities today. A team from the Texas Advanced Computing Center (TACC), the University of Texas Center for Transportation Research and the City of Austin believe that AI can help to optimize traffic flow.
Their work is developing searchable traffic analyses using deep learning and data mining. The tool uses the raw data generated from traffic cameras, with their algorithm capable of recognizing the various objects in the footage, and then characterizing how those objects move and interact. This information can then be queried by traffic planners to better understand the transport network.
“We are hoping to develop a flexible and efficient system to aid traffic researchers and decision-makers for dynamic, real-life analysis needs,” the team say. “We don’t want to build a turn-key solution for a single, specific problem. We want to explore means that may be helpful for a number of analytical needs, even those that may pop up in the future.”
Smarter traffic management
The system is capable of automatically tagging each object it encounters in the raw data, and then tracks their movements throughout the footage. By comparing outputs from each frame, the algorithm is able to discover relationships among the objects.
The system was put through it’s paces in two practical use cases. The first saw it count the number of vehicles travelling down a road, whilst the second identified near misses between vehicles and pedestrians. The results showed that the algorithm was around 95% accurate when counting the vehicles.
The system automatically counted vehicles in a 10-minute video clip, and preliminary results showed that their tool was 95 percent accurate overall. The team believe that the initial findings validate the system, which in turn will be crucial (and cost effective) in assessing traffic models in use today.
“Current practice often relies on the use of expensive sensors for continuous data collection or on traffic studies that sample traffic volumes for a few days during selected time periods,” they explain. “The use of artificial intelligence to automatically generate traffic volumes from existing cameras would provide a much broader spatial and temporal coverage of the transportation network, facilitating the generation of valuable datasets to support innovative research and to understand the impact of traffic management and operation decisions.”
Spotting near misses
The system was also capable of accurately spotting a number of near misses between vehicles and pedestrians. As none of these incidents were real-life dangers, they probably would go undetected in existing traffic monitoring systems, but they nonetheless help to identify dangerous locations and hopefully allow staff to intervene before injuries occur.
The team hope to continue developing the platform so that it can autonomously analyze other safety-related things, such as where pedestrians cross the road unsafely.
It’s a nice example of how AI can be effectively used to provide more comprehensive and accurate analysis of the kind of traffic data most cities are generating today to provide decision makers with actionable insights.
“The highly anticipated introduction of self-driving and connected cars may lead to significant changes in the behavior of vehicles and pedestrians and on the performance of roadways,” the team conclude. “Video data will play a key role in understanding such changes, and artificial intelligence may be central to enabling comprehensive large-scale studies that truly capture the impact of the new technologies.”
Check out the video below to see the system in action.

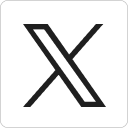



