Population displacement is a huge issue around the world, and indeed, involuntary migration was one of the major risks raised in the WEF’s Global Risks Report 2018. Around the world there has been no shortage of stories highlighting the challenges integrating large numbers of migrants into their host countries.
Researchers from Stanford believe they’ve developed an algorithm that can help raise the employment rates and overall integration of these migrants.
The work, which was documented in a recently published paper, saw historical data on refugee resettlement in both the US and Switzerland analyzed. This found that economic self-sufficiency required a range of things, such as the education level of the individual, their knowledge of English, and the location they settled in their new home country. This translated into some refugees having much higher chances of settling than others.
Smarter relocation
The algorithm was able to assign placements for refugees based upon this data, with the assignments giving them the best chance of integrating. Indeed, the researchers believe it increases their chances of finding a job by up to 70%.
“As one looks at the refugee crisis globally, it’s clear that it’s not going away any time soon and that we need research-based policies to navigate through it,” they say. “Our hope is to generate a policy conversation about the processes governing the resettlement of refugees, not just on the national level in the United States but internationally as well.”
Another attractive aspect of the algorithm is its ease of implementation. The team believe it can be installed very easily at virtually no cost and immediately begin to offer support to government agencies.
The technology solves an increasingly pressing problem, as huge numbers of refugees often overwhelm government agencies who then distribute them randomly, or at least according to local capacity rather than suitability.
“Our motivation was to bring the best of cutting-edge social science to an area of high policy priority that needs innovation but, because of the limited resources and challenges of navigating large numbers, has not been able to innovate from within,” the team say.
Training the system
The system was trained on data from over 30,000 refugees gleaned from a resettlement agency in the US. The data, which was collected over a 5 year period, included not only socioeconomic data on each refugee, but also where they were resettled and their eventual employment status.
The team put the system through its paces on a training set and was able to accurately predict the optimal location for refugees arriving in the United States in 2016. If the algorithm had been used to select the location for each refugee, the average employment rate would have been 41% higher.
The process was then replicated with refugees being resettled in Switzerland between 1999 and 2013. When the test was run again, this time they were able to increase the employment rate by 73% over the method actually used.
“The employment gains that we’re projecting are quite substantial, and these are gains that could be achieved with almost no additional cost to the governments or resettlement agencies,” the researchers say. “By improving an existing process using existing data, our algorithm avoids many of the financial and administrative hurdles that can often impede other policy innovations.”
Suffice to say, the work is at a very early stage in its development, so the authors are cautious about getting carried away with their findings. They certainly aren’t advocating replacing existing methods, but they do believe that it could be a useful decision support tool to augment the tools officers use today.
They’re confident however that the cost-effectiveness of their solution can see it make a significant impact very quickly. They’re hopeful that the project will bring much needed data robustness to a problem that has relied upon very un-scientific methods for too long.

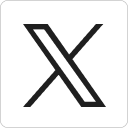



