Getting the right information, to the right people, at the right time can be vital in preventing the spread of deadly, yet wholly treatable diseases such as malaria and tuberculosis. That many of these diseases occur in poorer parts of the world where public health agencies are extremely strapped for cash makes this a considerable challenge however.
A team from USC Viterbi School of Engineering believe their algorithm can significantly help matters and cost effectively stop the spread of disease. It’s designed to make the most effective use of very limited resources.
The work, which was documented in a recently published paper, used a range of data, from behavioral and demographic data to epidemic disease trends to develop a model of disease spread that captures the population dynamics and contact patterns that underpins the spread of disease.
Modelling disease
These models were then used to power a number of computer simulations to test the algorithm on two real-world cases. The first of these was a TB outbreak in India, whilst the second was of gonorrhoea in the United States. In both scenarios, the algorithm was found to better utilize resources than the health policies of the time, by both identifying who the most at risk groups were, and better targeting messaging at them.
“Our study shows that a sophisticated algorithm can substantially reduce disease spread overall,” the authors say. “We can make a big difference, and even save lives, just by being a little bit smarter about how we use resources and share health information with the public.”
The model allowed the team to make better use of the resources available. It was found to concentrate heavily on certain groups, and even went as far as removing budget for certain groups with a high prevalence of the disease. It suggests the use of non-obvious patterns that may have eluded health officials.
By also taking into account realistic population dynamics, the team believe their work provides officials with more realistic tools than many existing algorithms for disease control.
“While there are many methods to identify patient populations for health outreach campaigns, not many consider the interaction between changing population patterns and disease dynamics over time,” they explain. “Fewer still consider how to use an algorithmic approach to optimize these policies given the uncertainty of our estimates of these disease dynamics. We take both of these effects into account in our approach.”
The team hope to expand their model to examine other infectious disease interventions, including the flu and HIV. It’s a clear indication of how AI can be used to make work not only smarter, but more effective.

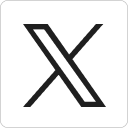



