AI has already proved to be pretty adroit at spotting early signs of disease in medical data. The latest project, from researchers at Georgia Tech, shows the potential of AI to spot early signs of heart failure. The research, which appears in the Journal of the American Medical Informatics Association (JAMIA), utilizes deep learning to examine data for temporality to provide earlier detections of incidents that can often lead to heart failure.
It’s an approach that is relatively unique in the application of artificial intelligence in healthcare, yet the researchers believe it could allow for much earlier detection of heart issues by identifying incidents that usually result in heart failure up to 18 months into the future.
Finding the connections
They can achieve this by looking for temporal relations among events that appear in our electronic medical records. It’s an approach that is often used in natural language processing, but much less so in deep learning. This allows them to better anticipate heart failure at its earliest stage, which will hopefully then allow them to deliver more effective preventative care to patients who are at high risk.
“Machine learning is being used in every aspect of health care. From diagnosis and treatments to recommendations for patient care after surgeries. This particular model is focused on deep learning, which has had great success in many industries. However, in health care, we are on the front of pioneering deep learning and Edward is one of the first ones to apply it,” the authors explain.
Whilst this is very preliminary work, it has already shown enough potential to make significant improvements on the existing models of prediction for a condition that affects 5.7 million adults in the United States alone, many of whom fail to get an early enough diagnosis to ultimately prevent premature death.
It’s also worth noting that this kind of predictive capability is capable from the relatively limited information available in our medical notes. If it was also able to learn from lifestyle data generated by ourselves, including diet, exercise and mental health, then it must surely be able to make more powerful predictions.
Nonetheless, it will be interesting to track the progress of the project as it attempts to find its way into the daily work of healthcare providers.

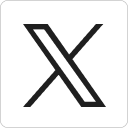



