Whilst previous years have seen an awful lot of discussion given to the potential of AI-based technology, this year has thus far seen a tremendous amount of effort given over to the ethical development of AI.
Very much in keeping with this trend was a report, published earlier this year, by the UK’s House of Lords, which made a number of principles around which they urge the development of AI to revolve:
- Artificial intelligence should be developed for the common good and benefit of humanity.
- Artificial intelligence should operate on principles of intelligibility and fairness.
- Artificial intelligence should not be used to diminish the data rights or privacy of individuals, families or communities.
- All citizens should have the right to be educated to enable them to flourish mentally, emotionally and economically alongside artificial intelligence.
- The autonomous power to hurt, destroy or deceive human beings should never be vested in artificial intelligence.
This, and many other similar publications have focused almost exclusively on the threat AI poses to society as a whole. That should not preclude us from exploring the threat AI poses to organizations themselves however.
That’s very much the message from Ajay Agrawal, Joshua Gans and Avi Goldfarb‘s latest book Prediction Machines.
“In addition to upside opportunities, AI poses systemic risks that may hit your business unless you take preemptory actions,” they explain. “Popular discussion seems to focus on the risks AI poses to humanity, but people pay much less attention to the dangers AI poses to organizations.”
They outline six key risks that they believe have to be understand and managed if AI is to benefit organizations:
- The risk that the predictions made by AI can lead to discrimination, even if inadvertently.
- The risk that insufficient data, both in terms of volume and quality, will render predictions also of poor quality. If we prescribe great confidence to these poor predictions then it can have severe consequences.
- The risk that hackers can inject incorrect input data in order to fool prediction machines.
- A diversity risk will likely see a trade-off between individual and system-level outcomes, such that lower diversity might benefit individual AI performance, but create higher risk of massive failure.
- By being open to interrogation, AI runs the risk of not only intellectual property theft but also of hackers identifying vulnerabilities in your system.
- Last, but not least, is a feedback risk that threatens to derail an AI-based system by helping it to learn destructive behavior.
Whether at an organizational or societal level, it’s important that the risks associated with AI are tackled, and it’s pleasing to see a growing number taking these risks seriously.

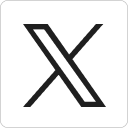



