For a period a few years ago, crowdsourcing was the next big thing, with a flurry of projects emerging off the back of James Surowiecki’s hit book about the wisdom of crowds. To an extent, the rise of AI has supplanted crowdsourcing and its drifted towards the rather less sexy mainstream. A recent study from a team at Iowa State University highlights how the two can effectively work together however.
Central to the success of most AI-driven projects is the ability to provide algorithms with high quality data so that they can train to spot the right patterns. Preparing that data can be time consuming, so the team turned to the crowd to perform the task. The crowd were tasked with helping the researchers develop an AI-system that can accurately identify the tassels of corn plants.
“This kind of automation could allow us to shave years off the time it would take to do this research otherwise,” the researchers explain. “This approach can help us breed more effective crop varieties faster.”
Recruiting the crowd
The team recruited a pool of volunteers from Amazon’s Mechanical Turk, each of whom were instructed to identify tassels in various images of corns. The identification was performed by drawing a square around the feature they believed to be a tassel. These labelled images would then be used to train the algorithm to do the job itself.
“You have to train a computer model to recognize the difference between, say, a leaf and a tassel,” the team explain. “One way to accomplish that is through machine learning, where you show instances of the object with a label over and over and the system builds its own rules to recognize that object on its own.”
The traditional process of labelling each of these images would involve graduate students or some other person with a degree of expertise in the given discipline. This latest study highlights the potential for people with minimal levels of expertise to do an equally proficient job. Whilst the results from the Mechanical Turk participants were slightly lower than those from trained experts, they were still of sufficient quality to train the algorithm.
Supporting plant science
The work is important because developing methods for automatically identifying tassels and other plant parts has a number of potential use cases in plant science. For instance, it can support research into understanding how weather affects tasseling, or understanding how various corn hybrids perform. What’s more, it allows this kind of work to be done much more quickly than existing methods.
The research also highlighted the value of inter-disciplinary teams, with researchers pulling in knowledge in areas such as statistics, bioinformatics, engineering and psychology. Whilst this made the work more complex as getting everyone on the same page was a challenge, it nonetheless contributed to the success in a major way.
If you’re interested in the use of microworkers from platforms such as Mechanical Turk for research purposes, you might enjoy a recent article on the value presented by such a workforce.

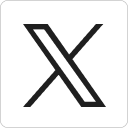



