The media has been awash with breathless prose about the capabilities of artificial intelligence in recent years. One would be forgiven for thinking that machines are practically at human levels of cognition already, or at least will be very soon.
A recent study from UCLA highlights just how far there still is to go. The study illustrated a number of quite significant limitations that the researchers believe we have to understand and improve upon before we let ourselves get carried away.
The researchers ran a number of experiments to test the progress made with machine vision. The first of these saw the VGG-19 deep learning network shown color images of various animals and objects, with each image altered in some way. For instance, a camel might have been doctored to have zebra stripes. Across 40 different images, it was only able to correctly identify the object five times.
Easily fooled
The researchers suggest that humans typically identify objects from their shape, but that AI-systems appear to be using a different method. This was highlighted in a second experiment where VGG-19 was shown images of glass figurines alongside a second network, called AlexNet.
Once again however, after being trained using images from the ImageNet database, both systems were unable to identify the glass figurines with any degree of accuracy. Similar results were achieved in a third experiment that tested the same two systems on a fresh set of images.
The goal was not so much to test the reliability of the systems as to understand how they identified the objects. This understanding was furthered by a fourth experiment where both networks were shown another bunch of images, all of which were solid black.
On this task, the AI did much better, producing the correct guess in their top five choices around half of the time. Still not great performance, but certainly better, and the authors reason that the AI did better with black objects because they lack ‘internal contours’, which the team believe confuse the AI.
A fifth, and final experiment then scrambled each image to make it more difficult to identify. The team picked six images that the AI had correctly identified in the previous experiments, and jumbled them up. Interestingly, whilst human volunteers struggled to identify the scrambled images, the computers did so five out of six times.
The team believe this happens because humans see the entire object when they look at it, whereas computers tend to look at fragments of it instead.
“This study shows these systems get the right answer in the images they were trained on without considering shape,” they continue. “For humans, overall shape is primary for object recognition, and identifying images by overall shape doesn’t seem to be in these deep learning systems at all.”
Given the number of computer vision systems entering the market, the team are optimistic that their work will do much to improve the quality and reliability of these systems.

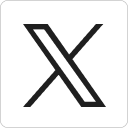



