On a recent trip to Accenture’s Dock facility in Dublin, they showcased an AI-based tool to recommend new recipes having crunched through thousands of existing recipes to come up with potential new flavors. Interesting though the work is, perhaps such an approach is far more interesting when applied to innovation itself.
A growing proportion of innovation today is what’s known as recombinative, and sees existing ideas and concepts applied in fresh and creative ways. Researchers are increasingly deploying AI-based tools to help devise some of these possible roads to explore.
For instance, a recent paper from researchers at Carnegie Mellon University and the Hebrew University of Jerusalem highlights an AI driven approach to mine databases of patents and research papers for ideas that can be recombined into solutions for new problems.
Central to the approach was an attempt to find analogies that connect seemingly disparate methods and problems. They used crowdsourcing to understand how people form analogies, before using this to train a deep learning algorithm to then mine the intellectual databases for potential innovations.
“After decades of attempts, this is the first time that anyone has gained traction computationally on the analogy problem at scale,” the authors say. “Once you can search for analogies, you can really crank up the speed of innovation, If you can accelerate the rate of innovation, that solves a lot of other problems downstream.”
Suffice to say, discovering analogies is not the easiest thing for a computer to do as it’s often one of those things we humans do without always understanding how we do it. Previous attempts at automating the job first required a handcrafted data structure to be created, which is incredibly time consuming and therefore not at all scalable.
Into the Crowd
The team turned to Mechanical Turk to recruit a number of volunteers to look for analogous products via the product innovation website Quirky. They did this by looking for a range of products with similar purposes or means of doing their job.
Each participant was told to explain how they came to their conclusions. For instance, they might describe a product in terms of both its purpose and its mechanism. This helps to provide connectors that can be used in creating the analogies.
These insights were then fed into the algorithm, before it was set loose on an additional dataset of product descriptions. The researchers tasked the algorithm with coming up some analogies of its own. Interestingly, it proved rather good at the task, and went beyond mere surface similarities to discover similarities between seemingly very different products. Indeed, when these were tested, the new product suggestions were rated as the most innovative ideas.
The team believe that the approach could easily be scaled up to be used as part of the innovation process by allowing organizations to find previously hidden connections between patents or research papers. With both datasets enormous and growing at a tremendous rate, it’s a problem that well suits autonomous methods, so it will be interesting to see what comes next for the team and their system.
A similar project was documented in a recently published paper in Nature. The researchers used a similar approach to derive connections between words and subjects, before trawling through around 3.3 million scientific abstracts from papers published between 1922 and 2018 on materials science.
The approach was able to capture fundamental knowledge within the field, such as the nature and structure of the periodic table and the way chemicals’ structures relate to their properties, whilst also suggesting a few new chemical compounds with properties similar to those of thermoelectric materials that hadn’t been examined before.
The team believe this approach could be a effective and efficient way of exploring new avenues for scientific research, and therefore accelerate the advance of science in various fields.

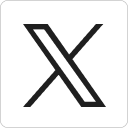



