With the amount of data produced by social networks, there is a clear temptation to mine that data and try and extrapolate trends or predictions for the future. Some of these efforts have proved quite successful, whether in predicting the spread of flu or on the next blockbuster movie. Predicting the viral qualities of content however has remained much more elusive.
Central to many of these attempts is what’s known as the sensors hypothesis, which questions whether it’s possible to find a group of people within a social network for whom their position allows them to monitor what information goes viral within that network. If that could be achieved, then it would be possible to predict the viral spread of content.
A study, conducted by researchers from the Universidad Autónoma of Madrid, the NICTA of Australia, and the American universities Yale and the University of California-San Diego, set out to test this very theory.
The researchers utilized the friendship paradox found on Twitter (amongst other places of course). This peculiar phenomenon reveals that your friends on Twitter nearly always have more friends than you do. When the team analyzed some 40 million users of the site, with 15 billion followers between them, they found that each user had an average of 25 followers. Each of those followers however had an average of 422 followers.
“This means that a person’s followers have a role in a social network that makes them very relevant when it comes to spreading or receiving information,” explains another of the researchers, Manuel García Herranz, of the Computer Engineering Department at the Universidad Autónoma of Madrid.
For this follow up study, they took a random selection of users, and assigned some of their followers the status of the sensor group. After monitoring content trends, they discovered that this sensor group played a much more significant role in content dissemination than was previously thought.
“We were really surprised. We thought the method would give us a few hours early warning, but instead it gave us several days, and sometimes even weeks or months,” says co-senior author of the authors, James Fowler, professor of medical genetics and political science at the University of California-San Diego (USA). For example, the sensor model predicted the “viral” rise of the hashtag “#Obamacare” as a Twitter trend, detecting it two months before it peaked on Twitter, and three months before it reached the highest number of Google searches with that name.
The researchers believe that data from just 50,000 Twitter users is enough to achieve this level of prediction. What’s more, it can be used in real time, about different topics, in different languages and in different geographical regions, thus opening up the model to an incredibly wide range of applications, whether in the commercial, political or social spheres.
The researchers do provide a caveat alongside their research. They state that the model cannot predict the spread of information surrounding a particular event. It can however do this for things such as social movements, or the movement of ideas from the niche to mainstream stages.
“We found that monitoring social media in this manner offers a whole new way of monitoring the global spread of information about all sorts of topics,” comments another one of the researchers, Nicholas Christakis, co-director of the Yale Institute for Network Science, USA. This is undoubtedly a new way of predicting the future by analyzing the data that circulates on the social networks.
All of which may finally begin to see us make sense of the huge amounts of social data that is being generated on a daily basis.

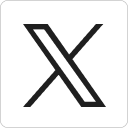



