The randomised control trial is the current gold standard in medical experimentation. It is far from perfect however. I wrote recently about the poor state of recruitment for such trials, and the recent attempts to crowdsource the matter and get more patients enrolled. After all, it’s been estimated that just 16% of cancer patients are even aware of suitable trials, let alone enrolled on any.
The closed nature of the trials themselves is also an issue, because the failed experiments are often as valuable as the successful ones, yet those failed trials seldom see the light of day. I’ve written before about the open notebook movement, and how they’re hoping to publicize trials in full, good and bad, in a bid to improve the learning possible from the whole process.
There are also issues surrounding repeatability of trials. There have been a number of accusations over the past few years about the poor replicability of research. In a RCT setting it is perhaps understandable, as human beings often behave very differently when not under trial conditions.
A collaboration between researchers at the Computation Institute and the University of Chicago is aiming to provide a boost to the sector. They are aiming to build a model that analyses and evaluates CommunityRx, which launched in 2012 with the aim of providing patients with a thorough report on managing their particular condition.
This could include information on local gyms, pharmacies and grocery stores, all in a personalized format to help support self-care in between visits to the doctor.
The hope is that the model will allow the team to assess the impact of the program in real time and in action, thus laying the foundation for a new way of testing healthcare programs in other areas.
“It’s very costly to try something in the real world and have it fail or be ineffective,” the researchers say. “So why not devote what amounts to a tiny fraction of resources in advance to consider and identify what might be helpful interventions? In the end, this could save a lot of money and provide enormously better programs that are much more effective.”
The researchers hope to better understand how the information provided to the patient about their condition then ripples out through their social networks, thus potentially providing a much wider impact than previously imagined.
“When patients take this information home, they might influence or share it with family members or friends who then might also change their perceptions about what’s available in the community,” they say. “We started looking for people with expertise to help us quantify and project the impact of a patient-level intervention on individuals, families, social networks, local communities and even the broader economy.”
It’s a similar approach to various studies over the past few years that have explored how all manner of things spread through social networks, from information to viruses. The agents in the model will be constructed to accurately reflect the patients currently using the service, with the behavioural characteristics of each agent fed by user surveys and qualitative data gathered at each intervention.
“The idea is to figure out how information flows through the system,” the developers say. “Surveys of patients and surveys of providers will help us generate networks of who’s talking to who, how many people they regularly talk to about health-related topics, and if they have talked to people about this program.”
Certainly an interesting approach, and it will be fascinating to see the results when they’re published. Is it an approach that could be used in other health programs?

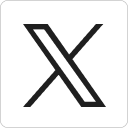



