The rise of mobile and social technologies in recent years has led to a deluge of data, much of which has been commandeered by researchers for their studies.
Whilst this data has obvious benefits, these benefits will only materialize if the quality of data can be guaranteed.
A team from the University of Twente have set out to provide an easy way of evaluating the quality of data generated by the crowd.
Validating crowd based data quality
Nowhere has this deluge been greater than in geographic information submitted by volunteers. When data is low quality and inconsistent it can bias analysis as the data does not accurately reflect the variable being studied. It’s important, therefore, to be able to quickly and accurately sift out inconsistent observations from the pack to ensure data remains scientifically viable.
The paper describes a novel automated workflow to do just that.
“Leveraging a digital control mechanism means we can give value to the millions of observations collected by volunteers” and “it allows a new kind of science where citizens can directly contribute to the analysis of global challenges like climate change” the authors say.
The process utilizes contextual information to judge the quality of the information, and has been built using a mixture of dimensionality reduction, clustering and outlier detection techniques.
The process was put through its paces on a project around the flowering of lilac plants in North America that relied heavily on volunteer data.
Whilst it’s inevitable that some unusual observations are valid, the authors showed that these outliers can still cause a bias in the trends. They suggest therefore, that identifying inconsistent observations is crucial to the accurate study of the topic and needs to be done from the outset.

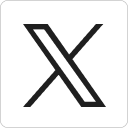



