Automated accounts are surprisingly common on Twitter, with applications ranging from nefariously influencing debates, to prodding us to be more charitable.
Stopping them has been an ongoing challenge however, and it’s one that was the focus of a recent DARPA competition to identify and squash bots that were being used to influence online discussions.
Bot-spotting
Twitter estimates that around 8-10% of its accounts are automated, but whilst many of these are harmless (if annoying), there are some that undertake more harmful work, whether it’s spreading propaganda or recruiting terrorists.
So, DARPA set out to try and find a better way of identifying the bots in their early days. A four week competition provided a clear winner and un-earthed some great strategies for tackling the bots.
The entrants in the competition were given live Twitter data during a debate on vaccinations in 2014. A number of bots were unleashed on the world during this period to try and influence the debate.
The aim was to test participants on whether they could identify which of the 7,000 or so accounts were actually one of the 39 bots created by DARPA. A correct guess earned a team 1 point, but an incorrect guess lost them 0.25 points.
A winning approach
The winning team were from social media analytics company Sentimetrix, who managed to discover the bots 12 days ahead of the deadline with just one incorrect guess. This placed them ahead of a team from the University of Southern California who took longer to discover the bots, but made no incorrect guesses.
So how did they do it? Well, the initial strategy was to try and identify the bots in the data, but none of the teams were able to automate this, and it ended up requiring a lot of manpower to do the job instead.
They utilized a pretrained algorithm in their hunt for bot behavior. The algorithm had been trained on data gained from the 2014 Indian election, where it was believed a lot of bots had been deployed to influence proceedings.
Telltale signs included unusual grammar and unusual behaviors for extended periods. This included things such as tweeting for the kind of prolonged spells that would be unusual or unlikely for human users.
Four accounts were immediately identified as bots, with these then used to help ID the others. For instance, it’s common for bot makers to use there existing accounts to link to new ones to therefore inflate their perceived popularity. This allowed clusters or networks to be identified.
Interestingly, flip-flopping behavior was also used to discover bots, as the team believed that bot makers could easily try and infiltrate one side of the debate, before then flipping and posting opposing arguments.
A key component of the Sentimetrix work was their visual dashboard that allowed humans to easily monitor the status of each user.
Eventually the team were able to detect another 25 bot accounts, which gave them sufficient data to update their algorithm and easily detect the remaining 10 bots.
With no clearly defined end point given to the teams, they could only stop when they believed that no more bots were on the loose.
Bot usage is undoubtedly on the rise, and with developers building ever more sophisticated accounts, it’s crucial that similar improvements are made in detection.
So challenges such as this, with the solutions then published online to assist further development, will only help matters, although of course, it’s also telling the bot makers the strategies being used by the hunters.
In that sense, it’s a cat and mouse battle that seems sure to endure.
If you liked this post, buy me a coffee

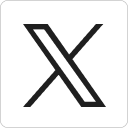




Hi, Adi!
My congratulations to Sentimetrix! These guys made the brilliant and useful job.
Hope there will be fewer bots at social networks.
Have a great day!
Interesting piece on Slate today about the use of Twitter bots by politicians.
http://www.slate.com/articles/technology/future_t…