Despite most health providers around the world using a range of tools and approaches to take a more holistic approach to healthcare, drugs remain a crucial part of the process.
Alas, their cost remains stubbornly high, with notorious cases such as Martin Shkreli highlighting the worst end of the spectrum.
A team from Carnegie Mellon have developed an automated experimentation system that they hope will determine the effect drugs have on proteins on a mass scale. They believe that the approach will reduce the number of experiments by up to 70%.
Reducing the cost of discovery
The approach, documented in a recent paper published in eLife, could potentially lead to a better and more accurate way of predicting how drugs will interact with their targets.
“Biomedical scientists have invested a lot of effort in making it easier to perform numerous experiments quickly and cheaply,” the authors say.
“However, we simply cannot perform an experiment for every possible combination of biological conditions, such as genetic mutation and cell type. Researchers have therefore had to choose a few conditions or targets to test exhaustively, or pick experiments themselves. The question is which experiments do you pick?”
Experimenting at scale
The paper suggests that a balance can be struck between the kind of experiments that can be confidently predicted, and those that can’t. This kind of judgement is something humans often struggle with as it requires the juggling of a huge number of potential outcomes concurrently.
To overcome this, the researchers used active learning, which is an application of machine learning. The approach requires the algorithm to repeatedly choose the experiments it wants to do and then learning from the patterns observed in the data.
The researchers tested their approach on synthetic data, before then allowing the machine to determine the experiments it wished to perform based upon the experience it had gained.
The experiments were then carried out using special liquid-handling robots and an automated microscope.
How it works
The algorithm began by studying the potential interactions between 96 drugs and 96 mammalian cell clones that had been tagged with different proteins.
From this, a total of 9,216 experiments were possible, with each of these consisting of the acquisition of images for a given cell clone when a particular drug was present.
The algorithm needed to learn how the proteins were affected in each experiment, without actually having to perform them all.
In the first round, images were collected for each clone, before those images were used to identify phenotypes that may (or may not) have been previously used to identify a characterized drug effect.
The algorithm then attempted to determine its own course by clustering images into phenotypes, which were then used to create a predictive model so that the algorithm could guess the outcome of thus far unmeasured experiments.
It performed this over 30 rounds, therefore completing 2,697 out of the 9,216 possible experiments, learning as it went and identifying additional phenotypes.
Getting bang for your buck
Using this approach, the algorithm was able to develop a model that was accurate 92% of the time, despite having only conducted 29% of the experiments.
“Our work has shown that doing a series of experiments under the control of a machine learner is feasible even when the set of outcomes is unknown. We also demonstrated the possibility of active learning when the robot is unable to follow a decision tree,” the authors conclude.
“The immediate challenge will be to use these methods to reduce the cost of achieving the goals of major, multi-site projects, such as The Cancer Genome Atlas, which aims to accelerate understanding of the molecular basis of cancer with genome analysis technologies.”

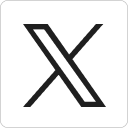




It certainly needs it, but I won't be holding my breath on this one.