I’ve written about a huge number of AI based applications in the past year or so that have utilized machine learning to perform all manner of seemingly miraculous feats.
This approach relies on huge amounts of data to train the machine to have a decent idea of how to respond in particular circumstances.
When these systems learn, it often involves the same bit of data being fed through the system multiple times. A recent study from Columbia University looks at how machines can forget what has come before, thus making it possible to develop more secure and private systems.
Machine Unlearning
The authors have developed a method whereby information can easily and rapidly be forgotten by a system, which they believe will be an increasingly important function for AI to possess.
“Effective forgetting systems must be able to let users specify the data to forget with different levels of granularity,” the authors say. “These systems must remove the data and undo its effects so that all future operations run as if the data never existed.”
As data becomes increasingly vast, the ability for systems to forget will be increasingly crucial as we attempt to maintain a degree of privacy. Whilst we are common with privacy threats with things like social network data, it is a less commonly appreciated issue when it comes to machine learning.
The authors reveal, however, that machine learning models deployed to deliver personalized medicines are leaking the genetic data of patients. It only takes a small amount of data for hackers to identify individuals from it.
Whilst most systems offer the ability to delete the raw data associated with a user, it’s generally not possible to delete the lineage of that data. This presents a significant problem to companies such as Google who must abide by data removal requests.
Marginal gains
The ‘machine unlearning’ approach works by converting machine learning systems such that they allow incremental updates to be performed in an efficient manner.
The method introduces an extra layer between the learning algorithm and the data it’s trained upon, which consists of a small number of summations. This is designed to eliminate any dependency each layer has on the other.
This allows the system to ‘unlearn’ a piece of data, and its lineage, without having to re-build the entire model and the associated relationships between data. By re-computing a small number of these, it’s possible to remove the data and its lineage completely from the system, and at a much faster rate than would currently be the case.
The team tested out their method in four systems currently operating in real-world environments across a range of scenarios, with positive results across all four. This will help them to take their work to the next level, whereby they hope to adapt the technique so it works more widely.
They’re confident however that ‘machine unlearning’ will play a key role in developing AI based systems that have both security and privacy at their core.
“We foresee easy adoption of forgetting systems because they benefit both users and service providers. With the flexibility to request that systems forget data, users have more control over their data, so they are more willing to share data with the systems. More data also benefit the service providers, because they have more profit opportunities and fewer legal risks,” they conclude.

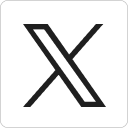



