I’ve written previously about how machine learning can be valuable in helping us find interesting avenues for research and exploration, with early forays into this field already happening in medicine.
A recent paper highlights how the approach should not be confined to medicine, with researchers highlighting its possible application in materials science.
“What we’ve done is show that, starting with a relatively small data set of well-controlled experiments, it is possible to iteratively guide subsequent experiments toward finding the material with the desired target,” the authors say.
They suggest that materials science has typically been driven by intuition, with experimentation largely a case of trial and error. As things get more complex however, the number of combinatorial possibilities makes this approach far from optimal.
Computational support
The researchers attempted to provide some computational support, with machine learning deployed to bring the algorithm rapidly up to speed. This process allowed a framework to be developed that helped to guide the next wave of experiments to be undertaken in the search for a new shape-memory alloy with low thermal hysteresis, which have a wide range of possible applications.
“The goal is to cut in half the time and cost of bringing materials to market,” they say. “What we have demonstrated is a data-driven framework built on the foundations of machine learning and design that can lead to discovering new materials with targeted properties much faster than before.”
Whilst such a machine learning based approach has seen traction in other fields, it’s believed to be one of the first attempts to use such a method in materials science.
Smarter science
Attempts thus far have largely focused on brute force examinations of databases. The team believe however that the interplay between structural, chemical and microstructural degrees of freedom presents too much complexity to be handled effectively by the current tools. What’s more, the experiments that are done tend to offer little feedback to guide future research.
The team suggest that their method can be applied to any materials class, or indeed any target property, which could prove crucial when complexity ramps up.

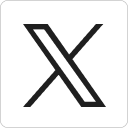



