Complex data is increasingly important to ecology and earth science work, and successfully dealing with such data can be a significant challenge for researchers who are not trained data scientists.
A recently published paper explores how machine learning can be used to help matters. The paper reveals that studies in these area often focus on the complex and challenging interactions between biotic and abiotic systems. Better understanding of these interactions helps us to understand natural systems better, and therefore make predictions about future behavior.
More traditional statistical methods are often far from ideal and unrealistic assumptions can be inferred from the data, with a resulting conclusion made that is anything but optimal. This is where the researchers believe machine learning can step in and help.
“A wider adoption of machine-learning methods in ecology and earth science has the potential to greatly accelerate the pace and quality of science,” they say. “Despite these advantages, however, machine-learning techniques have not met their full potential in ecology and earth science”.
Making machine learning more accessible
The researchers suggest that machine learning is not currently being used for this purpose due to a lack of collaboration between machine learning experts and the natural science community, and also a shortage of skilled people in the field.
They suggest that a greater level of financial support for both education and collaboration can help to overcome these issues.
“For many researchers, machine learning is a relatively new paradigm that has only recently become accessible with the development of modern computing. In this paper I suggest several mechanisms through which this useful method can be quickly introduced within the ecological and earth science fields, to ensure their wider application.” they say.
Another option is to utilize a platform such as the one I covered yesterday that is being developed by DARPA. Data-Driven Discovery of Models (D3M) aims to help in the development of automated means of crossing the data skills gap and allow non-experts to develop their own complex models.
It works in the same way as visual programming tools do in automating much of the complex data science work that underpins analysis, thus allowing less-skilled professionals to crunch big data.
Maybe the use of such a tool would be a useful short-cut whilst the funding and skills gap is worked on.

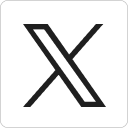



