Video games are a powerful way for AI to both learn and be put through its paces. Indeed, Google’s DeepMind famously illustrated this by testing its algorithms on 50 or so video games from the 80s, with tremendous success.
So it’s interesting to see the European startup Brightbox use gaming to train the neural networks at the heart of its latest driverless car technology.
Smart motoring
The company have history in car related products, with their Remoto product providing a range of services to allow ones car to be operated remotely. Their latest product, called Remoto Pilot, aims to provide automated driving services to motorists that can be retrofitted to existing cars.
Crucial to the technology is the use of stereo vision that works alongside vision algorithms that are trained on neural networks. These work with high definition maps and Global Navigation Satellite Systems to move us further towards autonomous driving.
The company used pre-computed datasets consisting of video samples of road situations that were used to train their algorithms. What makes it interesting however is that rather than just using real world footage, the team utilized footage from games such as Grand Theft Auto.
The images from the game (on the left) are used to inform the neural network what particular objects look like in a typical road scene. Annotated images (akin to those on the right) are then used to train the neural network on the locations of those objects.
This material is used alongside real world footage to provide the algorithms with a sizeable database of material to learn from. Suffice to say, this lends itself to a richer and more flexible neural network than is possible on a smaller training sample. The breadth and depth of circumstances possible when using virtual footage could play a big part in giving the algorithms the experience required to adapt to the unique circumstances found as the cars navigate our roads.
It’s an interesting approach, and you can see the fruits of their labor in the short demonstration video below.

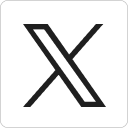



