The past few years have seen a number of interesting projects highlighting the potential for big data to unveil some fascinating insights into complex systems at a significantly faster speed than existing methods.
Whilst these results are indeed promising, a recent paper in Science highlights the work that still needs to be done before such methods can be widely relied upon.
The paper analyzed the reliability of a number of high profile automated systems, including the Global Data on Events Language and Tone (GDELT) and the International Crisis Early Warning System (ICEWS).
Reliability under the microscope
The researchers first set out to test just how reliable these systems were at predicting the various protests and other events they were designed to predict. Sadly, the answer seems to be rather weak, with few of the systems ever predicting the same events as the others.
The authors next tried to establish the validity of the systems. In other words, did the things they predicted would happen actually happen? Once again, the track record wasn’t great, with GDELT recording a 21% success rate.
A considerable problem with the systems was their reliance on news coverage of an event. If something wasn’t featured in the news then it wasn’t included in their databases, and this paucity of data can make predictions very unreliable.
“These global-monitoring systems can be incredibly valuable, transformative even,” the authors say. “Without good data, you can’t develop a good understanding of the world. But to gain the insights required to tackle global problems such as national security and climate change, researchers need more reliable event data.”
When is a protest not a protest?
A significant challenge for these kind of systems is in the classification of words. For instance, a news story featuring the word protest could be concerned with a relatively harmless event such as a politician protesting against a proposed policy.
“It’s so easy for us as humans to read something and know what it means,” the authors say. “That’s not so for a set of computational rules.”
The authors suggest a number of approaches to potentially improve such systems, including stronger communities between scholars. Key to this is transparency, with the authors recommending an open source approach to system development so that they can be built upon and improved. Alas, at the moment this is seldom the case, with much of the source materials protected by copyright.
Only if developers can test out their algorithms on accurate, open and representative datasets can we begin to accurately compare the various systems against one another. It’s possible that the kind of contests seen in the open innovation world could be a trigger for improvements.
There is still optimism around the potential for predictive big data, but at the moment work is still needed if they are to reach their potential in spotting epidemics, global warming and conflict escalation.

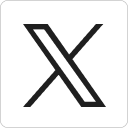



