The failures in coordination in the American security services have been widely reported in the aftermath of events like the 9/11 attacks, with the knowledge required to prevent the attacks available to staff but a lack of coordination resulted in this not reaching the people it should have, and preventable atrocities took place.
A recent paper set out to explore how AI can be used to improve the internal processes in the US State Department. The work was particularly interested in how the department can get better at correctly classifying the huge volume of emails generated each year internally.
Classifying the classified
The department is believed to generate around 2 billion emails per year, many of which contain classified information. Understanding, and thus correctly tagging, the content that needs classifying is a laborious job however.
The researchers used machine learning to improve this. They began by training their algorithms on around 1 million declassified cables from the 1970s between the State Department and overseas diplomats. Each message had been previously tagged as either secret, confidential, limited official use or unclassified.
Having trained the system, they set it to work to see if it could correctly classify documents, and especially whether it could correctly label content as deserving of classified status.
The algorithm proved particularly potent at doing this, with a 90% success rate in spotting classified content, and a false positive rate of just 11%. What’s more, the team believe that they could do even better with slightly better data to work with.
What makes something classified
Aside from the ability to classify content, the work also sheds a new light on the aspects of a message that most contribute to its security status. For instance, it emerged that the frequency of certain words was the best indicator of the security status of the overall message, with sender and recipient much less reliable.
Interestingly, some of the ‘false positive’ labels assigned by the machine, were actually proven to be human errors after all. In other words, they should have been classified but humans had tagged them otherwise.
It suggests that machines may play an increasingly important role in ensuring that content is classified correctly, but that for this to be effective they need to have good quality data on which to train themselves.
What’s more, the work also has the potential of revealing patterns in data sharing, and indeed in data removal within our security services that may in itself have security implications. After all, it emerged that classified content had a habit of going missing.
Whilst it’s undoubtedly interesting, it’s also clear that this is a very early stage of such a process, but given the billions spent each year by the State Department on classifying documents, it’s a work that hopefully merits further development.

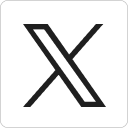



