As AI continues to develop at a rapid pace, a growing number of conversations turn to how we can ensure it retains its accountability. The broad consensus to date is that five core things are required:
- Someone responsible for each instance of AI
- The ability to explain what is done, how it’s done and why it’s done
- Confidence in the accuracy of the system, and knowledge of where biases may exist
- The ability for third parties to probe and audit the algorithms
- AI that is developed with fairness in mind
It’s perhaps natural to think that each of these tasks will be performed by human beings, but a recent study suggests that AI could do at least one of them for us.
Explaining yourself
The researchers developed an algorithm that is not only capable of performing its task, but also translates how it achieved it into reasonably understandable English via a documentation process that is performed at each stage of its work.
Suffice to say, it’s capability to do that is rather limited thus far, as it’s only capable of describing its work in recognizing human behavior in pictures (like the one above).
The algorithm trains itself using two distinct data sets. The first is to help it figure out what’s going on in the photo, and the second is to help it answer how it did so. The first half of this task is fairly standard, with a series of labelled images fed the algorithm. The second half however is quite novel, in that it utilizes three questions alongside each image, with 10 possible answers to each of them. So it might ask, “Is the person cycling? No, because… the woman doesn’t have a bicycle.”
This then gives it a degree of context around how it came to identify what was in the picture. It’s something the research team refer to as a ‘pointing and justification’ system’ in the sense of being able to justify any data that you care to point at.
Faith in the machine
It might appear quite modest beginnings, but it is nonetheless an important step, especially as algorithms are increasingly capable of learning on its own without any human input. The work done by companies such as DeepMind rely on this independent means of learning, but for the public to have faith in these systems, they will need a way of explaining what they do, and what’s more, explaining in a way that the end user rather than highly trained scientists can understand.
So this is an important first step, as traditionally, most ‘workings out’ have been long and complex strings of numbers that are incomprehensible to most of us. This is a good start in translating those strings into something more accessible.
The next stage is to take this approach and be able to apply it to a wider range of scenarios, and eventually develop a system that can operate in the kind of fuzzy scenarios we will see in domains such as driverless cars. In other words, it will require the machine to be able to explain for itself rather than humans to program explanations for it.

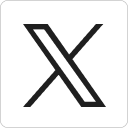



