Earlier this year I looked at a new report from the Urban Transport Group, which examined the role data was playing in the transformation of public transport.
With driverless cars likely to come to our cities within a decade, the amount of data generated by our urban transport systems is likely to mushroom considerably. Just as the vehicles become automated, so too will be the analytics that sits behind of, and indeed on top of all this data. This analytics will allow planners to discover trends and relationships that are simply too complex for humans.
Smart data
A good example of the kind of innovation we’re talking about is the Path to Park app, created by transportation company Parkeon. It uses AI to predict where drivers are most likely to find an available parking space by crunching live IoT data from parking meters.
The resulting application processes a massive volume (Gb/s) of IoT data from the millions of transactions coming from parking meters. The data is ultimately combined with geographical information from OpenStreetMap and used to predict the behavior of cars and traffic by building a real time model of each city that can analyze and predict ‘parking pressures’ on city streets.
Another example is Coyote, the European leader in real-time road information, who has developed an experimental machine learning data science platform that analyses anomalies in their IoT devices to improve the accuracy of road information for their 4.8 million users across Europe.
Coyote’s IoT devices and apps rely heavily on the accuracy of incoming data. In particular, driving speed limits within their embedded maps have to updated constantly with real-time data from other Coyote IoT devices.
The company used Machine Learning within Dataiku DSS to develop an algorithm that leverages vast amounts of IoT-derived data. The solution segments roads into sections and analyses patterns in each section. This enabled Coyote to build a predictive model that estimated the speed limit of the road section. The Machine Learning process facilitated the detection of speed limit anomalies and, consequently, enabled Coyote to estimate the global quality & reliability of the displayed speed limit.
The R&D project has increased speed limit reliability by 9% on a sample test, potentially resulting in more efficient and accurate real-time road information and travel time calculations for the 4.8 million Coyote users across Europe. In addition, thanks to the new platform’s focus on teamwork & cooperation, which enabled employees with differing skill-sets to work together, Data Mining & Visualization are now widespread within Coyote and there is a growing awareness of Smart Data issues.
IoT Data Science with Dataiku DSS
These solutions were made possible by the technology behind Dataiku (www.dataiku.com), maker of the collaborative data science software platform Dataiku Data Science Studio (DSS). Dataiku DSS makes it possible for organizations to reap the benefits of data science thanks to a collaborative interface for both expert and beginner analysts and data scientists. Dataiku offers a complete and accessible advanced analytics software platform that leverages all the tools and technologies required to develop a data research and development department that can build data-driven solutions to improve business.
Transport data is mushrooming, and the ability to make sense of that data in good time and cost effectively will be crucial in the successful management of our transport infrastructure. AI will play a crucial role in that, and the preceding examples provide a glimpse into what we are already doing, and what might be possible in future.

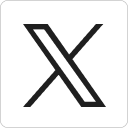



