Whilst we often believe automated systems to be purely rational entities, we’ve known for sometime that there are profound risks that such systems could hard-wire in many of the prejudices we ourselves have.
This risk is compounded by the difficulties many of us have in being aware of the various biases we ourselves have, so may develop algorithms believing we’re being much fairer than we really are.
Bias inherent in the system
“Questions about fairness and bias in machine learning are tremendously important for our society,” the authors say. “We have a situation where these artificial intelligence systems may be perpetuating historical patterns of bias that we might find socially unacceptable and which we might be trying to move away from.”
The study revolved around the Implicit Association Test, which is a common feature of social psychology research. It uses human response times to pair word concepts presented to each participant, with each person required to match up concepts they find similar.
For instance, ‘rose’ might be paired with ‘love’, whereas ‘spider’ might be paired with ‘ugly’. By monitoring the response time, the test uncovers our hidden assumptions.
The researchers developed an AI version of the Implicit Association Test, called GloVe. The algorithm at the heart of the program can represent the co-occurrence statistics of words in, say, a 10-word window of text. Words that usually appear near to one another have a stronger association than those that don’t.
Setting it loose
The system was set loose on text harvested from the Internet, with the text containing 840 billion words in total. The researchers had examined the text themselves, looking for biased associations, such as ‘engineer’ and ‘man’.
Interestingly, the machine picked out a number of well known human biases, along both gender and race. Indeed, the machine almost perfectly replicated the broad biases typically found in the Implicit Association Test over the many years of its use.
For instance, it associated male names with professional terms such as ‘salary’, whereas female names were associated with family related words, such as ‘wedding’.
Gender in the Valley
The issue takes on particular importance because of recent accusations labeled against Google that female staff are underpaid at the tech giant. There have been ongoing issues around the maleness of the sector, not just in terms of the tech companies themselves, but also the venture capital firms that bank roll them.
If a relatively homogeneous group are developing algorithms that underpin so much of our lives, how can we be sure that they aren’t hard-coding in biases?
The researchers suggest that developers might try and avoid this risk by the development of explicit, mathematics-based instructions for the machine learning programs underlying AI systems. This would work in a similar way to how parents try and instill fairness and equality in their children.
“The biases that we studied in the paper are easy to overlook when designers are creating systems,” they conclude. “The biases and stereotypes in our society reflected in our language are complex and longstanding. Rather than trying to sanitize or eliminate them, we should treat biases as part of the language and establish an explicit way in machine learning of determining what we consider acceptable and unacceptable.”

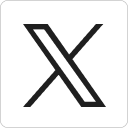



