The last few years have seen a growing range of technologies deployed to assist humanitarian efforts, whether it’s peacekeeping drones, crowdsourcing, or image analytics. With the rise in AI however, it’s perhaps no surprise that artificial intelligence can be utilized, and a recent paper describes how.
The paper uses AI to predict the gender of pre-paid mobile phone users with a high degree of accuracy. Rescue teams already use mobile phone data to help track those in need of assistance, but this new approach aims to go even further by helping to identify their gender, and therefore identify vulnerable groups such as women and children.
Making data rich
Whilst mobile phones are almost ubiquitous, in the developing world many are pre-paid, meaning that data often lacks key demographic identifiers. To overcome this, the team from Imperial College London developed a machine learning algorithm to analyze the phone data from 10,000 people. The analysis revealed a relatively easy and fail-safe way of determining the gender of the user, just from the data, with an accuracy rating of nearly 80%.
Phone data has been used in humanitarian crises such as the 2015 Nepal earthquake, with phone data used to track displaced populations so that aid can be delivered. This latest work builds on previous projects such as this to not only track users but also identify their gender and approximate age and social status.
It’s an approach that the team believe will enable more targeted relief efforts. For instance, the data could be used to identify groups of women or older people, who may be especially vulnerable in a crisis as they are more likely to have babies with them or be susceptible to disease.
“It is crucial to investigate how AI might help us mitigate some of the devastating effects of the world’s worst crises. Imagine if we could have used this technology in the aftermath of the Nepal crisis. We could have located refugee populations in search of help and determined who might’ve been the most vulnerable and needed assistance first,” the team say.
The team next hope to put their work to the test in the field, whilst also exploring whether it works as well in other locations.

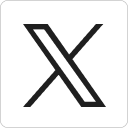



