Concussion has been headline news in recent months as a number of rugby players have suffered repeated blows to the head whilst on the pitch. It’s prompted a debate around player wellbeing and resulted in the traditional practice of returning to the field after a likely concussion being largely removed.
In the sporting context, there was a sense that caution was being erred on the side of when assessing players, but having a more accurate means of detecting both concussion, and the damage caused by it, would undoubtedly be invaluable.
Researchers believe that they’ve developed just such a method by utilizing AI to accurately detect brain damage, even years after the initial trauma occurred.
Effects of trauma
It’s estimated that up to 3.8 million concussions occur in the US alone each year, and whilst significant focus is given to the short-term effects, the long-term impact can be equally challenging. Longer-term issues can be hard to diagnose as it’s difficult to determine whether the symptoms were caused by the concussion or other neurological conditions (or even the aging process).
Currently, the only way to know for sure whether the brain has been damaged is to conduct a post-mortem examination. A method for diagnosing living patients does not exist.
The researchers recruited a cohort of former university athletes, with a section of the group having had known concussion during their career. Each volunteer was rigorously tested, including neuropsychological testing, genotyping, structural neuroimaging, magnetic resonance spectroscopy, and diffusion weighted imaging. The data was then aggregated and used to train the algorithm to detect differences between healthy and concussed athletes.
After crunching the data, the algorithm was able to detect concussion with 90% accuracy, due in large part by the identification of white matter connections between certain parts of the brain that suggested both degeneration in the brain, and also the brain’s attempt to compensate for the damage.
Upping the sample
The next step is to increase the size of the sample to ensure the algorithm is suitably robust. They also want to utilize magnetic resonance imaging (MRI) scanners to validate the signature in the hope that this will help to bring it to market and start diagnosing concussion in real life.
They also hope that the system will provide doctors with an accurate idea of what may be causing the symptoms exhibited by the patients. It’s an improvement that can’t come quickly enough.
“With 1.6 to 3.8 million concussions per year in the US alone, the prevalence of this injury is alarming,” the authors say. “It is unacceptable that no objective tools or techniques yet exist to diagnose them, not to mention the sheer lack of scientifically valid treatment options. With our work, we hope to provide help to the vast population of former athletes who experience neurological issues after retiring from contact sport.”
Future work will hopefully involve systematic comparisons with patient groups presenting with various other age-related neurological conditions, whilst also identifying potential new biomarkers for concussion to help refine the algorithms further.

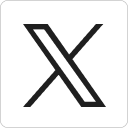



