Yesterday I looked at a new 4D camera that researchers believe could be invaluable in helping technologies such as autonomous vehicles navigate effectively in poor weather conditions.
Suffice to say, with the scale of this market, they are far from the only people taking an interest in this field. Researchers from the University of Adelaide in South Australia and Lund University in Sweden have recently published a paper in which they describe how the dragonfly has provided the inspiration for a new approach to autonomous driving.
At the heart of the project is a neuron within the brain of the dragonfly that allows it to anticipate the movement and trajectory of its prey. The neurons, known as Small Target Motion Detectors (STMD), increase the ability of the dragonfly to rapidly respond to the object they’re tracking. For instance, if the object goes behind something, then the neurons allow it to predict when and where it might reappear.
The researchers initially replicated these qualities in a miniature robot, and the plan is for this to be scaled up should the method prove successful.
“It is one thing for artificial systems to be able to see moving targets, but tracing movement so it can move out of the way of things is a really important aspect to self-steering vehicles,” the authors say.
Predicting behavior
Whilst this is believed to be the first time insects have been the inspiration for behavior predictions, it is a well-trodden topic in the autonomous vehicle world.
For instance, back in 2015 a team from the University of Illinois at Chicago, developed a system that can predict what the driver is about to do and then make corrective actions.
“Say you’re reaching for a piece of paper and your hand is bumped mid-reach — your eyes take time to adjust; your nerves take time to process what has happened; your brain takes time to process what has happened and even more time to get a new signal to your hand,” the researchers said.
The researchers put the process through the test in a scenario that analyzed the movements of participants as they interacted with a virtual desk. They were disrupted in their attempts to do so however, with the algorithm used to predict the intended action of the subject, even when that intent was disrupted and thus not followed through.
The algorithm was successful in predicting the intentions of people, and therefore how they would move. The hope is that when used in a car, this would keep the car moving in the way the driver intended.
Or you’ve got the project published earlier this year by researchers at George Mason University to help autonomous vehicles better understand what is a bicycle, and what its intentions are.
The algorithm they’ve developed forms a central part of what they call Deep3DBox, which is able to take a 2D image, identify road users within it, and then create a 3D box that surrounds each of them. It is also capable of determining the direction the vehicle is going in. When the algorithm was tested, it was able to correctly identify 89% of vehicles, but cyclists were another matter, with just 75% of bicycles identified, with much less success determining their direction.
Better scanning equipment and maps will no doubt help improve this statistic, but the inherent unpredictability of cyclists adds an extra layer of difficulty to the task.
“Bicycles are much less predictable than cars,” the authors say, “because it’s easier for them to make sudden turns or jump out of nowhere.”
As with most things, the more approaches we can take towards tackling a problem, but better the odds that we will succeed, so it seems inevitable that driverless vehicles will continue their seemingly unstoppable march.

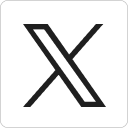



