Recently I looked at a fascinating piece of work undertaken in Australia to try and re-identify previously de-identified patient data. The authors found that patients can be relatively easily re-identified, usually without decryption.
The process used by the team involves linking the unencrypted parts of their record with information that is known about the individual. The results are similar to other studies, all of which show that seemingly mundane facts can be enough to isolate an individual, with a decrease in the precision of the data can reduce the risk of re-identification, albeit at the cost of the data’s utility.
This issue is especially important in areas such as healthcare, where the potential for data is so vast, yet the privacy concerns understandable given the sensitivity of the data we’re being asked to share.
Keeping data private
A recent paper from the University of Helsinki and Aalto University utilizes Bayesian reasoning to develop what the authors call differential privacy.
“Previously you needed one party with unrestricted access to all the data. Our new method enables learning accurate models for example using data on user devices without the need to reveal private information to any outsider,” the researchers explain.
The team tested out their privacy-aware model in data on cancer drug efficacy using gene expressions, with promising results.
“We have developed these methods with funding from the Academy of Finland for a few years, and now things are starting to look promising. Learning from big data is easier, but now we can also get results from smaller data,” the team say.

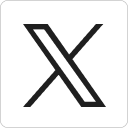



