Autonomous vehicles have certainly made tremendous progress, but you sense that the most difficult hurdles are still to be overcome as they make the transition from driving in test environments to unshackled motoring on public roads.
A recent paper outlines an integrated framework to help cars interact with each other and their environment more effectively.
“One of the main challenges associated with connected and automated driving is the lack of a systematic approach to integrate both vehicle connectivity and vehicle automation attributes for maximizing the performance benefits,” the authors say.
The framework combines digital, physical and social systems and appreciates that vehicles will inevitably operate at various levels of automation, with these levels dependent both upon the vehicle operator and the environment the vehicle is in. For instance, the passenger may switch on full automation for braking and steering operations whilst in a traffic jam, whilst another might prefer full control when driving in a built up area, but is happy with automation on the motorway.
The system is underpinned by a parallel learning theory that they believe will allow such distinct driving approaches to safely co-exist. The system will utilize machine learning to analyze the information about the vehicle, it’s driver and data about the action of driving. Each of these can be assessed and processed in parallel.
“The developed parallel driving framework is a ground-breaking approach in both short- and long-term, whose full realization in the long term requires systematic collaborative efforts from multidisciplinary sectors,” the team say.
The team are confident that they can roll out a simplified version of their cloud-based platform relatively quickly, and they expect to release a demonstration system that can showcase the functions and performance potentials early in 2018.

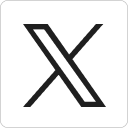



