The use of AI by HR departments is relatively low, but a few examples highlight the potential of the technology to help with talent management, albeit providing the data on performance is available.
A domain where performance data is readily available is in football, where stats are generated not only on appearances, goals and other match day data, but also on physical and mental attributes associated with a player. A recent study was able to use this data to identify players whose transfer fees represented good value versus those that were over-priced.
The researchers analyzed the salaries and performances of 6,082 professional football players using machine learning. Each player was analyzed according to 55 different attributes that were designed to reflect the skillset of the player. These included measurements related to performance (such as scoring and passing accuracy), behavior (such as aggression and vision), and abilities (such as speed, acceleration, and ball control). By combining the scores for each of these, the researchers believe they were able to accurately gauge what each player was worth in terms of salary, and by comparing them with their peers, whether they are worth it or not.
Spotting weak spots in the team
A second study has also trawled through the full array of data about Premier League football players to help managers identify potential weak spots in each team.
The study pored over data on player activity levels, including not only the distance they cover each match, but the intensity of that activity. This kind of analysis is not new, but the team wanted to fill in an important contextual gap by overlaying the data with tactical insight. This provides understanding not just of how they move, but why the players move. By providing this unique analysis for each position on the pitch, the researchers believe it provides a valuable insight into the performance of both individual players and the team as a whole.
The distance data was synchronized with video footage from each game, with the combination able to help construct a physical profile for each tactical role, both when that player has the ball and when they don’t. For example, when strikers have the ball they carried out specific, high-intensity efforts in the offensive part of the pitch, whereas full backs would do so when overlapping or running the channel. They would usually then attempt to cross the ball after this high-intensity burst.
When players didn’t have the ball, defensive players would perform their own high-intensity efforts when covering space or recovering their positions, with players across the team putting a lot of effort into closing opposition players down.
Player analyses
The researchers hope that their work can better inform managers about the physical and tactical performances of their players during matches. It adds an extra nuance to the way teams interpret their data.
They hope to further develop the system via a partnership with Liverpool John Moores University, which will result in a commercial product that can be offered to professional teams to monitor players. The system will utilize AI to pattern match in real time and provide a key context to tactical activities. They believe the system will allow managers to not only search out the weakest links in their own team, but also in their opposition.
Suffice to say, professional football, and perhaps sport in general, is relatively unique in terms of the amount of performance data created by athletes. This presents scientists with the opportunity to use AI tools like this to provide decision support to coaches. At the moment, HR managers don’t have anywhere near as much data available on the performance of staff, but these projects provide a glimpse into the possibilities should such data ever become available.

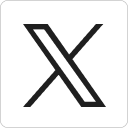



