The risk of AI systems hard-coding in the biases of their developers is one of the biggest challenges facing AI today. The size of the challenge was highlighted by recent work from MIT and Stanford University, which found that three commercially available facial-analysis programs display considerable biases against both gender and skin-types.
For instance, the programs were nearly always accurate in determining the gender of light-skinned men, but had an error rate of over 34% when it came to darker-skinned women.
The findings cast additional doubt on how AI systems are trained, and how accurate their suggestions actually are. For instance, the developers of one system that was analyzed claimed accuracy rates of 97%, but when the training data was examined, 77% of the faces were male, and 83% of them were white.
“What’s really important here is the method and how that method applies to other applications,” the authors say. “The same data-centric techniques that can be used to try to determine somebody’s gender are also used to identify a person when you’re looking for a criminal suspect or to unlock your phone. And it’s not just about computer vision. I’m really hopeful that this will spur more work into looking at [other] disparities.”
Facial recognition
The researchers analyzed three general-purpose facial-analysis systems. The systems are typically used to match faces in different photos whilst also identifying characteristics including age and gender.
The team collected a set of images that is more representative of society than traditional data sets used when training facial recognition systems. That meant they had many more women and people with dark skin than is normal. In total, the new dataset contained over 1,200 images.
Each of these images was then coded with the help of a dermatologic surgeon according to the Fitzpatrick scale of skin tones. This is a six-point scale from light to dark that was originally developed to assess risk of sunburn.
The data was then tested on three commercial facial-analysis systems to see just how accurate they were. Sadly, all three fared terribly when analysing female faces, and especially when the women had darker skin. Indeed, across the three systems, the error rate for this cohort was 20.8%, 34.5% and 34.7% respectively, with this jumping to 46.8% for the darkest-skinned women in the data set. In other words, it’s no better than a random guess.
“To fail on one in three, in a commercial system, on something that’s been reduced to a binary classification task, you have to ask, would that have been permitted if those failure rates were in a different subgroup?” the author concludes. “The other big lesson … is that our benchmarks, the standards by which we measure success, themselves can give us a false sense of progress.”
It’s a fascinating piece of work that highlights just how far such AI systems still have to go before they can be considered fair and just. It also underlines the need for better regulation so that systems that claim to be highly accurate are held to account for biases hard-coded into their working.
Check out the video below to learn more about the research.

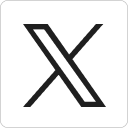



