As the number of applications of AI grows, it’s increasingly vital that it can be held to account, not only in terms of providing unbiased responses, but also accurate ones. A recent paper from Kyoto University highlights how AI can be adequately evaluated.
The method outlined in the paper provides a probability of the performance level of the algorithm based upon evaluation data fed to the test. For instance, it can provide a probability of reaching a particular level of accuracy.
“While reported statistics seem impressive, research teams and those evaluating the results come across two problems,” the authors say. “First, to understand if the AI achieved its results by chance, and second, to interpret applicability from the reported performance statistics.”
AI systems can only really be evaluated in a trustworthy way if there are an equal number of positive and negative results. If there is even the hint of bias one way or the other, it will tend to exaggerate the capabilities of the system.
Smarter analysis
To overcome this weakness, the paper outlines a new method that relies purely on the input data itself.
“The novelty of this technique is that it doesn’t depend on any one type of AI technology, such as deep learning,” the paper says. “It can help develop new evaluation metrics by looking at how a metric interplays with the balance in predicted data. We can then tell if the resulting metrics could be biased.”
The aim of the model, which has been made freely available, is to not only raise awareness of some of the weaknesses inherent in how we work with AI systems at the moment, but to also contribute to the development of better, more robust systems in the future.
“AI can assist us in understanding many phenomena in the world, but for it to properly provide us direction, we must know how to ask the right questions. We must be careful not to overly focus on a single number as a measure of an AI’s reliability,” the paper concludes.

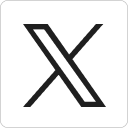



