Being able to predict potential problems in medical procedures is increasingly important, and technology is becoming more and more able to offer a helping hand. For instance, I wrote earlier this year about an AI-based approach to test for potential side effects of medicines, whilst last year an algorithm was developed to predict the likelihood of bone marrow complications.
It’s down this road that a team from Massachusetts General Hospital (MGH) have developed a system that can predict the risk of complications associated with mechanical ventilators. The work, which was documented in a recently published paper, performed more accurately than traditional methods that tend to rely on manual recording and intuition.
Predicting risk
“Ventilator-associated pneumonia is a very serious problem that is estimated to develop in up to half the patients receiving mechanical ventilator support,” the authors say. “Many patients die each year from ventilator-associated pneumonia, which can be prevented by following good patient care practices, such as keeping the head of the bed elevated and taking measures to prevent the growth of harmful bacteria in patients’ airways.”
Traditionally, staff aiming to assess risk would have to perform manual checks every 12 years of the ventilator settings as they’re adjusted during the day. The settings are designed to reflect the specific pressure required to keep the patient’s lungs open so that they can breath appropriately. Keeping them free from infection is crucial however as it can lead to ventilator-associated pneumonia.
“In our study, manual surveillance made many more errors than automated surveillance,” the researchers explain.
These errors often consist of things like false positives, misclassifications and general failure to detect potential cases. By contrast, the automated method had a 100% success rate, with the only caveat being that it required the necessary electronic data to perform so well.
These results were achieved over a three month period in early 2015 where the system was tested in four ICU units at MGH. During this period, a total of 479 patients received ventilator support. The automated system achieved 100% success rates across all three of the yellow, orange and red levels of risk that patients are vulnerable to. By contrast, the accuracy of humans during that period was just 40%, 89% and 70% respectively.
The initial study was followed by a second study conducted in another three month period in 2016. This saw another 431 patients receiving ventilator support, and again the automated system outperformed the expert staff. This second experiment saw an interruption in data that damaged performance, and this resulted in a monitoring system being developed to alert staff to such interruptions in future.
“An automated surveillance system could relieve the manual effort of large-scale surveillance, freeing up more time for clinicians to focus on infection prevention,” the authors conclude. “Automated surveillance is also much faster than manual surveillance and can be programmed to run as often as desired, which opens the way to using it for clinical monitoring, not just retrospective surveillance.”

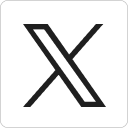



