The power of citizen science-based games has been something I’ve touched upon a number of times over the years, with them offering scientists the opportunity to engage thousands of people in a range of projects to significantly increase the speed of discovery. The latest of these was documented in a recently published paper, which described how Project Discovery has been used to classify cells.
As with previous uses of citizen scientists, the researchers found that the gamers helped to speed up the prediction of protein localization on a subcellular level. This improved the classification of these protein patterns, whilst also identifying 10 new members of the ‘Rods & Rings’ family of cellular structures for the first time.
This data is then being added to the Human Protein Atlas database that is used by researchers around the world to help them understand cells, proteins and disease development.
Building the game
The game, which was built in partnership with Massively Multiplayer Online Science and CCP Games, was played by over 300,000 players, who collectively generated around 33 million image classifications. It’s a total that is believed to be the biggest for any citizen science game. What’s more, the authors also believe that the citizen scientists performed comparably against an AI-based system that was designed to do a similar job.
The Localization Cellular Annotation Tool (Loc-CAT) is the first tool developed to annotate proteins and was designed to help with the studying of cells and their behavior. The AI tool outperformed the citizen scientists for many of the more common classes of protein, the human players did better for rare classes, and especially for classes that hadn’t been identified yet (and hence there was no training data to train the AI). The researchers believe that combining the citizen scientists and the AI they can boost performance yet further.
“I believe that the integration of scientific tasks into established computer games will be a commonly used approach in the future to harness the brain processing power of humans, and that intricate designs of citizen science games feeding directly into machine learning models has the power to rapidly leverage the output of large-scale science efforts,” they say. “We are grateful to all the citizen scientists who participated in this project, and for the discoveries they made.”

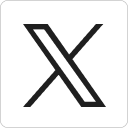



