Machine learning has become increasingly adept at drawing insights from large quantities of data, but can a similar process help to uncover the children most likely to be struggling at school? A recent study from the University of Cambridge suggests the answer is a definite yes.
The researchers were able to identify clusters of learning difficulties in 550 children that were not picked up in previous analyses of the children.
The children were recruited having been referred to the Centre for Attention Learning and Memory because they were struggling at school. The team were striving not just to include children who had already received a certain diagnosis, such as autism or ADHD, but who were struggling regardless of diagnosis. The aim was to allow them to spot any overlaps between diagnostic categories.
“Receiving a diagnosis is an important landmark for parents and children with learning difficulties, which recognises the child’s difficulties and helps them to access support. But parents and professionals working with these children every day see that neat labels don’t capture their individual difficulties – for example one child’s ADHD is often not like another child’s ADHD,” the authors say.
Machine insights
The team compiled a large amount of cognitive testing data from each child, which contained information on everything from spatial reasoning to listening skills. This dataset was used to train the algorithm, which was in turn able to suggest four clusters of difficulties for the children.
These clusters aligned well with various other bits of information on each child, including parental reports on any difficulties they had, and their school performance data. There was however, no correspondence with previous diagnoses.
To further examine things, 184 of the children had an MRI brain scan to see of the AI-driven groupings corresponded to any biological differences in the children. The groupings appeared to mirror patterns of connectivity in certain parts of the brain, which suggests the AI was able to spot differences that are reflected in the underlying biology of the child.
Two of the four groupings were in difficulties with working memory skills and difficulties processing sounds in words. The working memory issue has been linked previously with challenges with maths, whereas difficulties with processing sounds in words has been linked to challenges with reading.
“Past research that’s selected children with poor reading skills has shown a tight link between struggling with reading and problems with processing sounds in words. But by looking at children with a broad range of difficulties we found unexpectedly that many children with difficulties with processing sounds in words don’t just have problems with reading – they also have problems with maths,” the authors explain.
Overcoming difficulties
The team believe that their work will be useful in helping to go beyond any simple diagnostic labels and actually design better interventions that help children with various cognitive difficulties.
The study discovered a wide range of possible factors that underpin the difficulties a child is having, even among children struggling in the same subjects. It will hopefully be one step towards designing more effective and personalized interventions.
“These are interesting, early-stage findings which begin to investigate how we can apply new technologies, such as machine learning, to better understand brain function,” the team conclude.

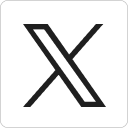



