Responding promptly and effectively to natural disasters is often extremely challenging, with poor resources compounding the challenges presented by the circumstances. A recent Stanford paper proposes the use of machine learning to help personnel better manage their resources.
It’s a sad reality of public life that many government agencies juggle priorities in an attempt to best allocate resources. The paper proposes using machine learning to help optimize this process by predicting where the resources will have the biggest impact.
The study was based upon the Clean Water Act, which regulates around 300,000 or so facilities. The lack of resources means that under 10% of these facilities are inspected per year.
Optimizing resources
As with previous machine learning projects, the team trained their algorithm on a large quantity of data from past inspections, with the algorithm then predicting the likelihood of an inspection failing, based upon a range of characteristics, including location, industry and inspection history. The model was tested on a range of facilities, including both those that have been previously inspected and those that have not.
The model created a risk score for each facility based upon the likelihood of failure, before adding real world context by applying four unique institutional constraints, including differing inspection budgets and frequencies.
In the (unlikely) scenario with the fewest constraints, the model was able to predict up to seven times as many violations compared to existing approaches. When the number of constraints rose, the number of violations was still twice those predicted by existing methods.
Feet on the ground
Despite the promising initial results, the team are keen to keep their feet on the ground. They rely on accurate data to perform effectively, and the data that they’re fed can be manipulated by agents keen to ensure they pass the inspection.
It also has to be remembered that many machine learning programs are developed in rarefied conditions rather than real-world ones, so their applicability and effectiveness might wane once real-world constraints are applied.
None of these challenges are insurmountable however, and the team are anxious to do more work to try and ensure that they don’t hold back what could be a very useful tool. They already plan to do additional work to explore the complexities of integrating machine learning technology into the Environmental Protection Agency’s enforcement work, whilst they are also exploring whether the same approach could be valuable in other contexts.
“This model is a starting point that could be augmented with greater detail on the costs and benefits of different inspections, violations and enforcement responses,” they say.

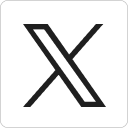



