Whilst it’s perhaps fair to say that AI hasn’t eaten the world yet, there have nonetheless been some interesting projects that have made valuable inferences from large data sets. The ability to make these inferences requires large qualities of data to get the algorithm up to speed, but of course not all organizations have such data sets at their disposal.
This has prompted researchers to experiment with simulated data to see if that can be as effective at training the system as real world data. This approach has provided fruitful early results, but does still nonetheless require a degree of time for the system to learn from the data its presented with.
Now, a team from Hong Kong Baptist University (HKBU) have teamed up with Tencent to create a new technique that speeds this aspect up, whilst maintaining accuracy levels. The work, which was documented in a recently published paper, reveals that two popular deep neural networks were trained in just four minutes and 6.6 minutes respectively, which is half the time previously taken for the AlexNet and ResNet-50 respectively.
Visual testing
Both neural networks are built on the ImageNet visual recognition system that contains a large-scale dataset. The tests enabled each system to accurately recognize and then label objects in particular photos. That this was achieved significantly faster than all previous records is what undoubtedly sets this approach apart however.
“We have proposed a new optimised training method that significantly improves the best output without losing accuracy. In AI training, researchers strive to train their networks faster, but this can lead to a decrease in accuracy. As a result, training machine-learning models at high speed while maintaining accuracy and precision is a vital goal for scientists,” the researchers say.
The improvement in training speed was achieved via improvements in both computing time and communication time. Computational efficiency was enhanced by using a simpler method known as FP16 to replace a more complex method, known as FP32. The communication process was enhanced by using a technique known as ‘tensor fusion’ to combine small chunks of data into larger pieces, and thus optimise the transmission pattern, and ultimately the efficiency of communication during the training.
The team believe their approach can be successfully adopted in various large-scale classification projects, or indeed other AI applications, including machine translation and natural language processing. It will be interesting to see whether it becomes widely adopted within the industry.

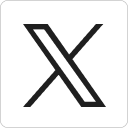



