As artificial intelligence has become more powerful, more and more attention is given to how we can ensure it’s as fair as possible. A recent project by researchers at MIT CSAIL suggests that the key might be to focus on how the data that underpins AI today is collected.
“Computer scientists are often quick to say that the way to make these systems less biased is to simply design better algorithms,” the researchers say. “But algorithms are only as good as the data they’re using, and our research shows that you can often make a bigger difference with better data.”
The research was able to not only identify data that could cause potential problems, but also to quantify the impact that factor could have on accuracy levels. They then used this to show how different ways of collecting data could reduce the various types of bias they had identified, whilst still maintaining high levels of predictive accuracy.
“We view this as a toolbox for helping machine learning engineers figure out what questions to ask of their data in order to diagnose why their systems may be making unfair predictions,” the team explain.
Fairer data
I wrote recently about a project that was aiming to provide comparable levels of predictive accuracy with a lot less training data. It’s a nice example of how more data is not always beneficial to the performance of the system. This could be because the additional data is of poor quality, but it could also be because it lacks fundamental diversity.
The team believe that their approach allows developers to look at datasets and easily see if biases exist, and whether more data is needed from particular demographic groups to make the data representative, and therefore fairer.
“We can plot trajectory curves to see what would happen if we added 2,000 more people versus 20,000, and from that figure out what size the dataset should be if we want to have the best of all worlds,” they say. “With a more nuanced approach like this, hospitals and other institutions would be better equipped to do cost-benefit analyses to see if it would be useful to get more data.”
The researchers believe this is perhaps the best way to improve the fairness of AI, as whilst you could request additional information from your existing pool of participants, this could simply result in acquiring largely irrelevant information.
As AI does become more powerful and plays a bigger role in the decisions we make in life, it’s positive to see a growing number of projects not only attempt to make those algorithms as fair as possible. Whilst this project suggests the need for authentic data, there is a growing usage of virtual data in healthcare use cases, especially in areas where real data might be limited. Hopefully this study will act as a reminder on the need for that data to result in fair outcomes, and not embed bias and discrimination.

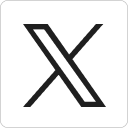



