The applications of machine learning in medicine and healthcare have been enormous, but one of the most interesting has been in the field of drug development. AI has been deployed to both find potential molecules to explore, and even to do the testing itself, whilst also helping with clinical trials, and even the reporting of side effects and progress in patients.
The latest effort in this field comes from researchers at the University of Cambridge, who have developed an algorithm for drug discovery that they believe is around twice as effective as the current industry standard. The work, which was documented in a recently published paper,
The work has already identified four new molecules that activate a protein that is believed to be important in understanding diseases such as schizophrenia and Alzheimer’s. The team focused specifically on being able to predict whether a molecule will activate certain physiological processes. It’s an area that’s hamstrung by a lack of data to work with.
“Machine learning has made significant progress in areas such as computer vision where data is abundant,” the researchers explain. “The next frontier is scientific applications such as drug discovery, where the amount of data is relatively limited but we do have physical insights about the problem, and the question becomes how to marry data with fundamental chemistry and physics.”
Smarter drug discovery
The Cambridge team worked in partnership with Pfizer to develop a model that can separate pharmacologically relevant chemical patterns from ones that aren’t relevant. Perhaps most importantly, the system is able to examine molecules that are both active and inactive, before identifying those that are most important for drug action.
The system uses a mathematical principle known as random matrix theory, which provides it with predictions about the various statistical properties of a seemingly random dataset. This can then be compared against statistics of known active/inactive molecules to understand the chemical patterns that are most important for binding.
This approach allows the team to determine the key chemical patterns not only from the molecules they’ve found that are active, but also from those that aren’t. This allows them to gain vital insights from failed experiments just as much as successful ones. It’s an approach that in testing allowed them to identify four new molecules that activated the CHRM1 receptor, which is a protein believed to have a key role to play in both Alzheimer’s and schizophrenia.
“The ability to fish out four active molecules from six million is like finding a needle in a haystack,” the researchers say. “A head-to-head comparison shows that our algorithm is twice as efficient as the industry standard.”

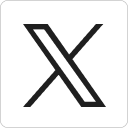



