Turberculosis (TB) is a pretty nasty disease that’s caused by the Mycobacterium turberculosis bacteria and it primarily affects the lungs. It’s difficult to spot as it often doesn’t really have any symptoms, and this latent form of the disease can effect a huge number of people. Despite only 10% of latent infections progressing to the active disease, this active form still effects over 10 million people per year, with 1.6 million of them dying from the disease.
Even if treated, it is possible for the condition to return, at which point it is usually treated with antibiotics. Being able to predict those most prone to recurrence of the disease is vital to successful treatment, and researchers from the University of Michigan believe that machine learning could play a role.
In a recently published paper, they describe an AI-based diagnostic tool that could help to identify TB patients with the greatest risk of reactivation of the disease.
“A multi-array test can provide a more detailed, disease specific glimpse into patient’s infection and likely outcome,” the authors explain. “Using a precision medicine approach reveals previously obscured diagnostic signatures and reactivation risk potential.”
Predicting reactivation
Current tests for latent TB involve a skin scratch test or a blood test. These are able to identify single biomarkers but usually can’t distinguish between memory immune responses, vaccine-initiated responses and non TB mycobacteria exposure. As such, successful identification is currently under 5%.
What’s more, if antibiotics are used unnecessarily, it increases the likelihood of antibiotic resistance developing. The tool developed by the team aims to identify patients most at risk reactivation to overcome this challenge.
The tool utilizes multiple biomarker assays in each blood test, which the researchers believe significantly increases the odds of successfully diagnosing TB in the patient.
The team believe that their tool can be both cost-effective and scalable, but it perhaps goes without saying that it’s some way from the market at the moment. It does nonetheless provide another interesting example of how AI could be used in healthcare.

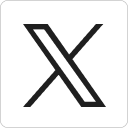



