The effectiveness of surveillance cameras in both deterring and catching crime is significantly undermined by the challenges involved in monitoring and locating criminals in the act. It’s a hugely labor intensive job that can be akin to finding a needle in a haystack. A number of projects have attempted to use AI to improve matters, by using algorithms to monitor live footage for behaviors that look suspicious so that humans can then investigate.
For instance, a few years ago a British startup called ThirdEye attempted to monitor CCTV footage and alert security personnel when they detected suspicious behavior.
“CCTV captures thousands of potentially useful clips everyday, such as thefts, assaults, mugging and people collapsing on the street from medical emergencies. However, due to the lack of manpower, we currently cannot analyse this footage. It’s a problem that irritates me. I worked in my Dad’s wholesale warehouse and at Buckingham Palace and felt powerless to see stock that I put out being stolen or being frisked when leaving work to make sure that I hadn’t taken anything. My co-founder actually built CCTV software to detect copper cable thieves- it was a huge problem with hundreds of people losing out on power when these were stolen,” co-founder Raz Ghafoor said after their launch.
Alas, the project didn’t take off and the company has folded, but that hasn’t stopped others trying to follow in their footstep. The latest project of this ilk comes from a team from Binghamton University, who have developed technology to detect suspicious activity in surveillance footage. The work, which was documented in a recently published paper, consists of a tracking algorithm, known as Kerman (Kernelized Kalman filter).
The system utilizes single board computers that are mounted onto surveillance cameras to help process the footage and identify particular actions and behaviors that warrant further attention. It perhaps goes without saying that such work requires significant computing power, and the team get around that by utilizing decentralized computing platforms that distribute the workload across multiple computing nodes rather than a centralized server.
This means that the video doesn’t need to be transferred to a central location, therefore making the system considerably more agile, with data processing times reduced and faster decisions made.
Whilst the system monitors feeds, it doesn’t identify, track or record any of the activities, therefore ensuring that privacy is maintained. The team believe that future innovations in hardware will enable performance levels to improve still further, and provide an even more effective tool for security professionals to use. It’s fair to say that we’re still at a very early stage in the development of these kind of systems, but they do nonetheless provide an interesting insight into the direction of travel.

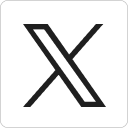



