The various crowd-based games that allow players to contribute to the advance of science at the same time as playing an enjoyable game is something I’ve touched upon numerous times, with research showing how beneficial these games have been to scientific progress.
Arguably the most well known of these games is Eterna, which aims to help in the design of new RNA molecules. With hundreds of thousands of players, it has attracted a huge audience, but what lessons can be learned from the way those players play the game? That was the question posed by a team from the Stanford University School of Medicine, who wanted to see if they could build an AI-based system that could understand the most effective way to play the game based upon the experiences of the human players.
The work, which was documented in a recently published paper, examined around 1.8 million design choices proposed by Eterna players, before utilizing the EternaBrain neural network that was designed to predict the choices of the most effective players. They built upon this algorithm to try and develop a system that could perform at a similar or higher level than the current Eterna champions.
“Our findings suggest that it should be possible to create automatic algorithms for computer RNA design that emulate or outperform human RNA designers,” the researchers say. “But we’re not there yet; we still have a lot to learn from both gamers and AI researchers.”
We’ve seen numerous attempts by AI researchers to develop algorithms to play video games better than human players can in the past, and whilst there is a possible research benefit in doing so, it’s hard to escape the apparently trivial nature of the endeavor.
At least the work on EternaBrain are using these advances to help power the science of RNA design forward, and the team believe their approach could also be used on the numerous other crowdsourced science games, or the more complex challenges being tackled by Eterna players, such as the design of RNA computers and 3D machines.

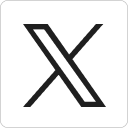



