Clickbait is one of those aspects of the web that has become pervasive as publishers have looked to build the audience that is so crucial to sustaining their advertising revenues. Given the propensity of its use, it’s likely that humans have become pretty good at spotting a clickbait article from something more substantial. Can technology do a similar, or even better job?
That was what research from Penn State set out to explore, with both humans and an algorithm tasked with creating the best clickbait they could muster. These headlines were then used to train a detection algorithm to successfully spot clickbait when it encountered it.
Lo and behold, it proved to be rather good, and was around 14.5% better at doing so than other systems. The team believe that their method may have use beyond the realm of clickbait detection.
“This result is quite interesting as we successfully demonstrated that machine-generated clickbait training data can be fed back into the training pipeline to train a wide variety of machine learning models to have improved performance,” they say. “This is the step toward addressing the fundamental bottleneck of supervised machine learning that requires a large amount of high-quality training data.”
Data challenges
The results in detecting clickbait are promising, not least because of the relative paucity of clearly labeled data. This rendered training the algorithm difficult, and this is an area the researchers believe needs to improve for future work.
The nuance with which clickbait is deployed adds an additional layer of complexity to the task, as it comes in many different forms that can make spotting it challenging.
“There are clickbaits that are lists, or listicles; there are clickbaits that are phrased as questions; there are ones that start with who-what-where-when; and all kinds of other variations of clickbait that we have identified in our research over the years,” the researchers explain. “So, finding sufficient samples of all these types of clickbait is a challenge. Even though we all moan about the number of clickbaits around, when you get around to obtaining them and labeling them, there aren’t many of those datasets.”
Different approaches
It was also interesting to observe the fundamentally different approaches taken by the humans and the AI in detecting clickbait creation. For instance, those created by humans tended to have determiners in them, which include words such as ‘which’ and ‘that’.
Those with a training in journalism would also tend to use longer words and pronouns more often than other participants. Numbers were also more frequently used to begin headlines created by trained journalists.
The researchers hope to use the findings to guide their further exploration into the creation of automated fake-news detection systems.
“For us, clickbait is just one of many elements that make up fake news, but this research is a useful preparatory step to make sure we have a good clickbait detection system set up,” they explain.

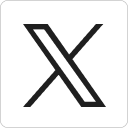



