Data clearly has tremendous potential for driving innovation and change within a business, but not all organizations are capable of utilizing data in the same way. A recent study from Wharton explores how different organizational structures influence how data spurs innovation.
The authors remind us that companies are getting less bang for their innovation buck than they used to, and so they wanted to explore why the heavy investment in data and analytics is not resulting in more innovation.
They focus specifically on the structure of collaboration networks that sit at the heart of innovation. Does data work best when those networks are densely structured (around a specific facility for instance), or dispersed? Both are widely used in corporate innovation initiatives, but these structures do appear to have an impact on the way these organizations innovate.
Creating recombination
I’ve written a number of times in the past about the increasingly recombinative nature of innovation, and data analytics can be a great help in achieving that recombination of ideas and technologies. Analytics can connect those dots, and the research found works especially well in decentralized innovation environments.
The advantage of a decentralized structure is that small teams work on the problem and as a result really understand the problem. It gives them a clearer insight than those operating in a more centralized structure. Whilst a decentralized structure lacks the coordination of its centralized peer, the use of analytics can help to connect and coordinate their work and therefore reap the benefits of recombination.
There have been numerous approaches to this task, perhaps most notably by researchers at Carnegie Mellon University and the Hebrew University of Jerusalem who used an AI-driven approach to mine databases of patents and research papers for ideas that can be recombined into solutions for new problems.
Central to the approach was an attempt to find analogies that connect seemingly disparate methods and problems. They used crowdsourcing to understand how people form analogies, before using this to train a deep learning algorithm to then mine the intellectual databases for potential innovations.
“After decades of attempts, this is the first time that anyone has gained traction computationally on the analogy problem at scale,” the authors say.
“Once you can search for analogies, you can really crank up the speed of innovation,” they continue. “If you can accelerate the rate of innovation, that solves a lot of other problems downstream.”
The potential is clear, and whilst the Wharton team don’t reveal whether this kind of analytics-supported decentralized innovation is enough to overcome the productivity challenges highlighted in the data, it is perhaps an approach worth pursuing.

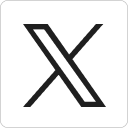



