One of the most common strategies for optimizing forecasting is the ‘wisdom of crowds’, which sees predictions aggregated to form a collective forecast. The idea is that by pooling expertise together, the outlying predictions cancel themselves out, and a sensible consensus is reached.
New research from the University of Tokyo aims to deploy a similar trick for improving the power and accuracy of existing algorithms that aim to forecast the future. They believe that by combining the various suboptimal predictions, they were able to provide a consensus prediction that was better than the best forecasts. They believe their system could provide valuable early warnings for everything from economic shocks to natural disasters.
A changing picture
In time series data, the graph typically fluctuates according to changes in the data, whether it’s representing stock prices or water levels. The complexity of these systems makes predictions very difficult to achieve, as fluctuations can be wild and seemingly unpredictable.
A common approach used to make sense of this kind of data is known as delay embedding, which takes a sequence of observations and embeds them into a higher-dimensional space. This allows the current value to be combined with evenly spaced values from the past.
The challenge is that selecting the dimension to be embedded, and the delay lag you assign to it is mostly a case of trail and error in the search for the optimal combination. The researchers have used AI to optimize the selection process, and they believe this new approach is significantly better than existing methods.
“We found that the ‘wisdom of the crowd,’ in which the consensus prediction is better than each on its own, can be true even with mathematical models,” they say.
The method was tested on real-world flood data, but they believe it to be applicable in a wide range of scenarios. Forecasting is increasingly important in areas as diverse as neuroscience and fluid dynamics, so they’re confident in the applicability across a number of use cases.

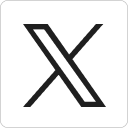



